Open Access
ARTICLE
ENSOCOM: Ensemble of Multi-Output Neural Network’s Components for Multi-Label Classification
Department of Information Systems, Al-Qunfudhah Computing College, Umm Al-Qura University, Al-Qunfudhah, Mecca, Saudi Arabia
* Corresponding Author: Khudran M. Alzhrani. Email:
Computers, Materials & Continua 2022, 72(3), 5459-5479. https://doi.org/10.32604/cmc.2022.028512
Received 11 February 2022; Accepted 18 March 2022; Issue published 21 April 2022
Abstract
Multitasking and multioutput neural networks models jointly learn related classification tasks from a shared structure. Hard parameters sharing is a multitasking approach that shares hidden layers between multiple task-specific outputs. The output layers’ weights are essential in transforming aggregated neurons outputs into tasks labels. This paper redirects the multioutput network research to prove that the ensemble of output layers prediction can improve network performance in classifying multi-label classification tasks. The network’s output layers initialized with different weights simulate multiple semi-independent classifiers that can make non-identical label sets predictions for the same instance. The ensemble of a multi-output neural network that learns to classify the same multi-label classification task per output layer can outperform an individual output layer neural network. We propose an ensemble strategy of output layers components in the multi-output neural network for multi-label classification (ENSOCOM). The baseline and proposed models are selected based on the size of the hidden layer and the number of output layers to evaluate the proposed method comprehensively. The ENSOCOM method improved the performance of the neural networks on five different multi-label datasets based on several evaluation metrics. The methods presented in this work can substitute the standard labels representation and predictions generation of any neural network.Keywords
Cite This Article
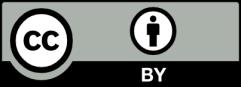