Open Access
ARTICLE
Compact Bat Algorithm with Deep Learning Model for Biomedical EEG EyeState Classification
1 Department of Computer Science, College of Computer and Information Sciences, Prince Sultan University, Saudi Arabia
2 Department of Computer Sciences, College of Computer and Information Sciences, Princess Nourah Bint Abdulrahman University, Riyadh, 11671, Saudi Arabia
3 Department of Information Systems, College of Science & Art at Mahayil, King Khalid University, Saudi Arabia
4 Department of Computer Sciences, College of Computing and Information System, Umm Al-Qura University, Saudi Arabia
5 Department of Computer and Self Development, Preparatory Year Deanship, Prince Sattam bin Abdulaziz University, AlKharj, Saudi Arabia
6 Department of Natural and Applied Sciences, College of Community-Aflaj, Prince Sattam bin Abdulaziz University, Saudi Arabia
* Corresponding Author: Anwer Mustafa Hilal. Email:
Computers, Materials & Continua 2022, 72(3), 4589-4601. https://doi.org/10.32604/cmc.2022.027922
Received 28 January 2022; Accepted 01 March 2022; Issue published 21 April 2022
Abstract
Electroencephalography (EEG) eye state classification becomes an essential tool to identify the cognitive state of humans. It can be used in several fields such as motor imagery recognition, drug effect detection, emotion categorization, seizure detection, etc. With the latest advances in deep learning (DL) models, it is possible to design an accurate and prompt EEG EyeState classification problem. In this view, this study presents a novel compact bat algorithm with deep learning model for biomedical EEG EyeState classification (CBADL-BEESC) model. The major intention of the CBADL-BEESC technique aims to categorize the presence of EEG EyeState. The CBADL-BEESC model performs feature extraction using the ALexNet model which helps to produce useful feature vectors. In addition, extreme learning machine autoencoder (ELM-AE) model is applied to classify the EEG signals and the parameter tuning of the ELM-AE model is performed using CBA. The experimental result analysis of the CBADL-BEESC model is carried out on benchmark results and the comparative outcome reported the supremacy of the CBADL-BEESC model over the recent methods.Keywords
Cite This Article
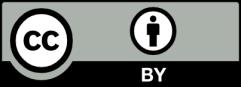
This work is licensed under a Creative Commons Attribution 4.0 International License , which permits unrestricted use, distribution, and reproduction in any medium, provided the original work is properly cited.