Open Access
ARTICLE
Enhancing Collaborative and Geometric Multi-Kernel Learning Using Deep Neural Network
1 Department of Mechatronics and Control Engineering, University of Engineering and Technology, Lahore, 54890, Pakistan
2 Department of Information Sciences, Division of Science and Technology, University of Education, Lahore, 54000, Pakistan
3 Riphah School of Computing & Innovation, Faculty of Computing, Riphah International University, Lahore Campus, Lahore, 54000, Pakistan
4 Department Software, Gachon University, Seongnam, 13120, Korea
* Corresponding Author: Allah Ditta. Email:
Computers, Materials & Continua 2022, 72(3), 5099-5116. https://doi.org/10.32604/cmc.2022.027874
Received 27 January 2022; Accepted 08 March 2022; Issue published 21 April 2022
Abstract
This research proposes a method called enhanced collaborative and geometric multi-kernel learning (E-CGMKL) that can enhance the CGMKL algorithm which deals with multi-class classification problems with non-linear data distributions. CGMKL combines multiple kernel learning with softmax function using the framework of multi empirical kernel learning (MEKL) in which empirical kernel mapping (EKM) provides explicit feature construction in the high dimensional kernel space. CGMKL ensures the consistent output of samples across kernel spaces and minimizes the within-class distance to highlight geometric features of multiple classes. However, the kernels constructed by CGMKL do not have any explicit relationship among them and try to construct high dimensional feature representations independently from each other. This could be disadvantageous for learning on datasets with complex hidden structures. To overcome this limitation, E-CGMKL constructs kernel spaces from hidden layers of trained deep neural networks (DNN). Due to the nature of the DNN architecture, these kernel spaces not only provide multiple feature representations but also inherit the compositional hierarchy of the hidden layers, which might be beneficial for enhancing the predictive performance of the CGMKL algorithm on complex data with natural hierarchical structures, for example, image data. Furthermore, our proposed scheme handles image data by constructing kernel spaces from a convolutional neural network (CNN). Considering the effectiveness of CNN architecture on image data, these kernel spaces provide a major advantage over the CGMKL algorithm which does not exploit the CNN architecture for constructing kernel spaces from image data. Additionally, outputs of hidden layers directly provide features for kernel spaces and unlike CGMKL, do not require an approximate MEKL framework. E-CGMKL combines the consistency and geometry preserving aspects of CGMKL with the compositional hierarchy of kernel spaces extracted from DNN hidden layers to enhance the predictive performance of CGMKL significantly. The experimental results on various data sets demonstrate the superior performance of the E-CGMKL algorithm compared to other competing methods including the benchmark CGMKL.Keywords
Cite This Article
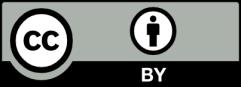
This work is licensed under a Creative Commons Attribution 4.0 International License , which permits unrestricted use, distribution, and reproduction in any medium, provided the original work is properly cited.