Open Access
ARTICLE
Frequency Domain Adaptive Learning Algorithm for Thoracic Electrical Bioimpedance Enhancement
1 Department of Electronics and Communication Engineering, Koneru Lakshmaiah Education Foundation, K L University, Vaddeswaram, Guntur, 522502, Andhra Pradesh, India
2 Department of Computer Science and Engineering, Faculty of Science and Technology, ICFAI Foundation for Higher Education, Hyderabad, 500082, Telangana, India
3 Department of Electrical, Electronics and Communication Engineering, GITAM Institute of Technology, GITAM Deemed to be University, Visakhapatnam, 530045, Andhra Pradesh, India
* Corresponding Author: Md Zia Ur Rahman. Email:
Computers, Materials & Continua 2022, 72(3), 5713-5726. https://doi.org/10.32604/cmc.2022.027672
Received 23 January 2022; Accepted 08 March 2022; Issue published 21 April 2022
Abstract
The Thoracic Electrical Bioimpedance (TEB) helps to determine the stroke volume during cardiac arrest. While measuring cardiac signal it is contaminated with artifacts. The commonly encountered artifacts are Baseline wander (BW) and Muscle artifact (MA), these are physiological and non-stationary. As the nature of these artifacts is random, adaptive filtering is needed than conventional fixed coefficient filtering techniques. To address this, a new block based adaptive learning scheme is proposed to remove artifacts from TEB signals in clinical scenario. The proposed block least mean square (BLMS) algorithm is mathematically normalized with reference to data and error. This normalization leads, block normalized LMS (BNLMS) and block error normalized LMS (BENLMS) algorithms. Various adaptive artifact cancellers are developed in both time and frequency domains and applied on real TEB quantities contaminated with physiological signals. The ability of these techniques is measured by calculating signal to noise ratio improvement (SNRI), Excess Mean Square Error (EMSE), and Misadjustment (Mad). Among the considered algorithms, the frequency domain version of BENLMS algorithm removes the physiological artifacts effectively then the other counter parts. Hence, this adaptive artifact canceller is suitable for real time applications like wearable, remove health care monitoring units.Keywords
Cite This Article
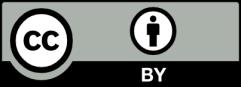