Open Access
ARTICLE
An Efficient Stacked-LSTM Based User Clustering for 5G NOMA Systems
1 Faculty of Engineering, Multimedia University, Cyberjaya, 63100, Malaysia
2 School of Information Technology, Monash University, Subang Jaya, 47500, Malaysia
* Corresponding Author: Chee Keong Tan. Email:
Computers, Materials & Continua 2022, 72(3), 6119-6140. https://doi.org/10.32604/cmc.2022.027223
Received 12 January 2022; Accepted 18 March 2022; Issue published 21 April 2022
Abstract
Non-orthogonal multiple access (NOMA) has been a key enabling technology for the fifth generation (5G) cellular networks. Based on the NOMA principle, a traditional neural network has been implemented for user clustering (UC) to maximize the NOMA system’s throughput performance by considering that each sample is independent of the prior and the subsequent ones. Consequently, the prediction of UC for the future ones is based on the current clustering information, which is never used again due to the lack of memory of the network. Therefore, to relate the input features of NOMA users and capture the dependency in the clustering information, time-series methods can assist us in gaining a helpful insight into the future. Despite its mathematical complexity, the essence of time series comes down to examining past behavior and extending that information into the future. Hence, in this paper, we propose a novel and effective stacked long short term memory (S-LSTM) to predict the UC formation of NOMA users to enhance the throughput performance of the 5G-based NOMA systems. In the proposed strategy, the S-LSTM is modelled to handle the time-series input data to improve the predicting accuracy of UC of the NOMA users by implementing multiple LSTM layers with hidden cells. The implemented LSTM layers have feedback connections that help to capture the dependency in the clustering information as it propagates between the layers. Specifically, we develop, train, validate and test the proposed model to predict the UC formation for the futures ones by capturing the dependency in the clustering information based on the time-series data. Simulation results demonstrate that the proposed scheme effectively predicts UC and thereby attaining near-optimal throughput performance of 98.94% compared to the exhaustive search method.Keywords
Cite This Article
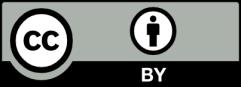