Open Access
ARTICLE
WDBM: Weighted Deep Forest Model Based Bearing Fault Diagnosis Method
1 Department of Computer Science, City University of Hong Kong, Hong Kong, 999077, China
2 School of Computer and Software Engineer, Xihua University, Chengdu, 610039, China
3 Nanjing University of Aeronautics and Astronautics, Nanjing, 210008, China
4 McGill University, Montreal, H3G 1Y2, Canada
* Corresponding Author: Letao Gao. Email:
Computers, Materials & Continua 2022, 72(3), 4741-4754. https://doi.org/10.32604/cmc.2022.027204
Received 12 January 2022; Accepted 08 March 2022; Issue published 21 April 2022
Abstract
In the research field of bearing fault diagnosis, classical deep learning models have the problems of too many parameters and high computing cost. In addition, the classical deep learning models are not effective in the scenario of small data. In recent years, deep forest is proposed, which has less hyper parameters and adaptive depth of deep model. In addition, weighted deep forest (WDF) is proposed to further improve deep forest by assigning weights for decisions trees based on the accuracy of each decision tree. In this paper, weighted deep forest model-based bearing fault diagnosis method (WDBM) is proposed. The WDBM is regard as a novel bearing fault diagnosis method, which not only inherits the WDF’s advantages-strong robustness, good generalization, less parameters, faster convergence speed and so on, but also realizes effective diagnosis with high precision and low cost under the condition of small samples. To verify the performance of the WDBM, experiments are carried out on Case Western Reserve University bearing data set (CWRU). Experiments results demonstrate that WDBM can achieve comparative recognition accuracy, with less computational overhead and faster convergence speed.Keywords
Cite This Article
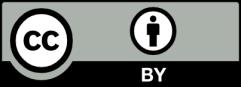
This work is licensed under a Creative Commons Attribution 4.0 International License , which permits unrestricted use, distribution, and reproduction in any medium, provided the original work is properly cited.