Open Access
ARTICLE
Crop Yield Prediction Using Machine Learning Approaches on a Wide Spectrum
1 Department of Electronics and Communication Engineering, Vel Tech Rangarajan Dr. Sagunthala R&D Institute of Science and Technology, Chennai, 600062, India
2 Department of Electrical and Electronics Engineering, Sri Venkateswara College of Engineering, Sriperumbudur, 602117, India
3 Suranaree University of Technology, Nakhon Ratchasima, 30000, Thailand
4 University of Central Punjab, Lahore, 54000, Pakistan
5 Government College University, Lahore, 54000, Pakistan
6 Virtual University of Pakistan, Islamabad Campus, 45550, Pakistan
* Corresponding Author: Worawat Lawanont. Email:
Computers, Materials & Continua 2022, 72(3), 5663-5679. https://doi.org/10.32604/cmc.2022.027178
Received 12 January 2022; Accepted 08 March 2022; Issue published 21 April 2022
Abstract
The exponential growth of population in developing countries like India should focus on innovative technologies in the Agricultural process to meet the future crisis. One of the vital tasks is the crop yield prediction at its early stage; because it forms one of the most challenging tasks in precision agriculture as it demands a deep understanding of the growth pattern with the highly nonlinear parameters. Environmental parameters like rainfall, temperature, humidity, and management practices like fertilizers, pesticides, irrigation are very dynamic in approach and vary from field to field. In the proposed work, the data were collected from paddy fields of 28 districts in wide spectrum of Tamilnadu over a period of 18 years. The Statistical model Multi Linear Regression was used as a benchmark for crop yield prediction, which yielded an accuracy of 82% owing to its wide ranging input data. Therefore, machine learning models are developed to obtain improved accuracy, namely Back Propagation Neural Network (BPNN), Support Vector Machine, and General Regression Neural Networks with the given data set. Results show that GRNN has greater accuracy of 97% (R² = 0.97) with a normalized mean square error (NMSE) of 0.03. Hence GRNN can be used for crop yield prediction in diversified geographical fields.Keywords
Cite This Article
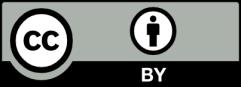
This work is licensed under a Creative Commons Attribution 4.0 International License , which permits unrestricted use, distribution, and reproduction in any medium, provided the original work is properly cited.