Open Access
ARTICLE
Evolutionary Algorithsm with Machine Learning Based Epileptic Seizure Detection Model
1 Department of Computer and Self Development, Preparatory Year Deanship, Prince Sattam bin Abdulaziz University, 16278, AlKharj, Saudi Arabia
2 Department of Computer Science, College of Science and Arts, King Khalid University, Mahayil, Asir, 62529, Saudi Arabia
3 Department of Information Systems, College of Computer and Information Sciences, Princess Nourah Bint Abdulrahman University, Riyadh, 11671, Saudi Arabia
4 Department of Computer Sciences, College of Computer and Information Sciences, Princess Nourah Bint Abdulrahman University, Riyadh, 11671, Saudi Arabia
5 Department of Natural and Applied Sciences, College of Community - Aflaj, Prince Sattam bin Abdulaziz University, 16278, Saudi Arabia
* Corresponding Author: Manar Ahmed Hamza. Email:
Computers, Materials & Continua 2022, 72(3), 4541-4555. https://doi.org/10.32604/cmc.2022.027048
Received 09 January 2022; Accepted 04 March 2022; Issue published 21 April 2022
Abstract
Machine learning (ML) becomes a familiar topic among decision makers in several domains, particularly healthcare. Effective design of ML models assists to detect and classify the occurrence of diseases using healthcare data. Besides, the parameter tuning of the ML models is also essential to accomplish effective classification results. This article develops a novel red colobuses monkey optimization with kernel extreme learning machine (RCMO-KELM) technique for epileptic seizure detection and classification. The proposed RCMO-KELM technique initially extracts the chaotic, time, and frequency domain features in the actual EEG signals. In addition, the min-max normalization approach is employed for the pre-processing of the EEG signals. Moreover, KELM model is used for the detection and classification of epileptic seizures utilizing EEG signal. Furthermore, the RCMO technique was utilized for the optimal parameter tuning of the KELM technique in such a way that the overall detection outcomes can be considerably enhanced. The experimental result analysis of the RCMO-KELM technique has been examined using benchmark dataset and the results are inspected under several aspects. The comparative result analysis reported the better outcomes of the RCMO-KELM technique over the recent approaches with the of 0.956.Keywords
Cite This Article
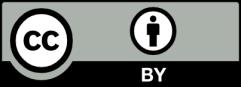
This work is licensed under a Creative Commons Attribution 4.0 International License , which permits unrestricted use, distribution, and reproduction in any medium, provided the original work is properly cited.