Open Access
ARTICLE
Multi-Scale Attention-Based Deep Neural Network for Brain Disease Diagnosis
1 Faculty of Information Technology, College of Computer Science and Technology, Beijing Artificial Intelligence Institute, Beijing University of Technology, Beijing, 100124, China
2 Department of Natural and Computing Science, University of Aberdeen, Aberdeen, U.K
* Corresponding Author: Yin Liang. Email:
Computers, Materials & Continua 2022, 72(3), 4645-4661. https://doi.org/10.32604/cmc.2022.026999
Received 08 January 2022; Accepted 02 March 2022; Issue published 21 April 2022
Abstract
Whole brain functional connectivity (FC) patterns obtained from resting-state functional magnetic resonance imaging (rs-fMRI) have been widely used in the diagnosis of brain disorders such as autism spectrum disorder (ASD). Recently, an increasing number of studies have focused on employing deep learning techniques to analyze FC patterns for brain disease classification. However, the high dimensionality of the FC features and the interpretation of deep learning results are issues that need to be addressed in the FC-based brain disease classification. In this paper, we proposed a multi-scale attention-based deep neural network (MSA-DNN) model to classify FC patterns for the ASD diagnosis. The model was implemented by adding a flexible multi-scale attention (MSA) module to the auto-encoder based backbone DNN, which can extract multi-scale features of the FC patterns and change the level of attention for different FCs by continuous learning. Our model will reinforce the weights of important FC features while suppress the unimportant FCs to ensure the sparsity of the model weights and enhance the model interpretability. We performed systematic experiments on the large multi-sites ASD dataset with both ten-fold and leave-one-site-out cross-validations. Results showed that our model outperformed classical methods in brain disease classification and revealed robust inter-site prediction performance. We also localized important FC features and brain regions associated with ASD classification. Overall, our study further promotes the biomarker detection and computer-aided classification for ASD diagnosis, and the proposed MSA module is flexible and easy to implement in other classification networks.Keywords
Cite This Article
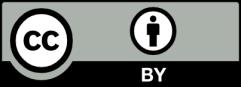
This work is licensed under a Creative Commons Attribution 4.0 International License , which permits unrestricted use, distribution, and reproduction in any medium, provided the original work is properly cited.