Open Access
ARTICLE
Printed Surface Defect Detection Model Based on Positive Samples
1 School of Computer Science and Artificial Intelligence, Changzhou University, Changzhou, Jiangsu, 213164, China
2 Focusight Technology Co, Changzhou University, Changzhou, Jiangsu, 213164, China
3 West Liberty University, 208 University Drive, West Liberty, 26074, USA
* Corresponding Author: Wang Hongyuan. Email:
Computers, Materials & Continua 2022, 72(3), 5925-5938. https://doi.org/10.32604/cmc.2022.026943
Received 07 January 2022; Accepted 14 March 2022; Issue published 21 April 2022
Abstract
For a long time, the detection and extraction of printed surface defects has been a hot issue in the print industry. Nowadays, defect detection of a large number of products still relies on traditional image processing algorithms such as scale invariant feature transform (SIFT) and oriented fast and rotated brief (ORB), and researchers need to design algorithms for specific products. At present, a large number of defect detection algorithms based on object detection have been applied but need lots of labeling samples with defects. Besides, there are many kinds of defects in printed surface, so it is difficult to enumerate all defects. Most defect detection based on unsupervised learning of positive samples use generative adversarial networks (GAN) and variational auto-encoders (VAE) algorithms, but these methods are not effective for complex printed surface. Aiming at these problems, In this paper, an unsupervised defect detection and extraction algorithm for printed surface based on positive samples in the complex printed surface is proposed innovatively. We propose a kind of defect detection and extraction network based on image matching network. This network is divided into the full convolution network of feature points extraction, and the graph attention network using self attention and cross attention. Though the key points extraction network, we can get robustness key points in the complex printed images, and the graph network can solve the problem of the deviation because of different camera positions and the influence of defect in the different production lines. Just one positive sample image is needed as the benchmark to detect the defects. The algorithm in this paper has been proved in “The First ZhengTu Cup on Campus Machine Vision AI Competition” and got excellent results in the finals. We are working with the company to apply it in production.Keywords
Cite This Article
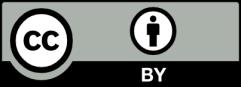