Open Access
ARTICLE
Two-Dimensional Projection-Based Wireless Intrusion Classification Using Lightweight EfficientNet
1 School of Strategic and Global Studies, Universitas Indonesia, Depok, 16424, Indonesia
2 National Institute of Information and Communications Technology (NICT), Koganei, 184-8795, Japan
3 Department of Electrical Engineering and Information Technology, Universitas Gadjah Mada, Yogyakarta, 55281, Indonesia
4 School of Computing, Korea Advanced Institute of Science and Technology (KAIST), Daejeon, 34141, Korea
* Corresponding Author: Muhamad Erza Aminanto. Email:
Computers, Materials & Continua 2022, 72(3), 5301-5314. https://doi.org/10.32604/cmc.2022.026749
Received 04 January 2022; Accepted 02 March 2022; Issue published 21 April 2022
Abstract
Internet of Things (IoT) networks leverage wireless communication protocols, which adversaries can exploit. Impersonation attacks, injection attacks, and flooding are several examples of different attacks existing in Wi-Fi networks. Intrusion Detection System (IDS) became one solution to distinguish those attacks from benign traffic. Deep learning techniques have been intensively utilized to classify the attacks. However, the main issue of utilizing deep learning models is projecting the data, notably tabular data, into an image. This study proposes a novel projection from wireless network attacks data into a grid-based image for feeding one of the Convolutional Neural Network (CNN) models, EfficientNet. We define the particular sequence of placing the attribute values in a grid that would be captured as an image. Combining the most important subset of attributes and EfficientNet, we aim for an accurate and lightweight IDS module deployed in IoT networks. We examine the proposed model using the Wi-Fi attacks dataset, called the AWID2 dataset. We achieve the best performance by a 99.91% F1 score and 0.11% false-positive rate. In addition, our proposed model achieved comparable results with other statistical machine learning models, which shows that our proposed model successfully exploited the spatial information of tabular data to maintain detection accuracy.Keywords
Cite This Article
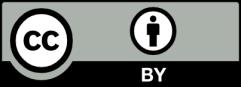
This work is licensed under a Creative Commons Attribution 4.0 International License , which permits unrestricted use, distribution, and reproduction in any medium, provided the original work is properly cited.