Open Access
ARTICLE
Deobfuscating Mobile Malware for Identifying Concealed Behaviors
Soongsil University, Seoul, 06978, Korea
* Corresponding Author: Haehyun Cho. Email:
Computers, Materials & Continua 2022, 72(3), 5909-5923. https://doi.org/10.32604/cmc.2022.026395
Received 24 December 2021; Accepted 16 March 2022; Issue published 21 April 2022
Abstract
The smart phone market is continuously increasing and there are more than 6 billion of smart phone users worldwide with the aid of the 5G technology. Among them Android occupies 87% of the market share. Naturally, the widespread Android smartphones has drawn the attention of the attackers who implement and spread malware. Consequently, currently the number of malware targeting Android mobile phones is ever increasing. Therefore, it is a critical task to find and detect malicious behaviors of malware in a timely manner. However, unfortunately, attackers use a variety of obfuscation techniques for malware to evade or delay detection. When an obfuscation technique such as the class encryption is applied to a malicious application, we cannot obtain any information through a static analysis regarding its malicious behaviors. Hence, we need to rely on the manual, dynamic analysis to find concealed malicious behaviors from obfuscated malware. To avoid malware spreading out in larger scale, we need an automated deobfuscation approach that accurately deobfuscates obfuscated malware so that we can reveal hidden malicious behaviors. In this study, we introduce widely-used obfuscation techniques and propose an effective deobfuscation method, named ARBDroid, for automatically deobfuscating the string encryption, class encryption, and API hiding techniques. Our evaluation results clearly demonstrate that our approach can deobfuscate obfuscated applications based on dynamic analysis results.Keywords
Cite This Article
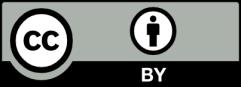
This work is licensed under a Creative Commons Attribution 4.0 International License , which permits unrestricted use, distribution, and reproduction in any medium, provided the original work is properly cited.