Open Access
ARTICLE
Intelligent Sign Language Recognition System for E-Learning Context
1 Department of Computer Science, Air University, Islamabad, 44000, Pakistan
2 Department of Computer Science, College of Computer, Qassim University, Buraydah, 51452, Saudi Arabia
3 Department of Computer Science and Software Engineering, Al Ain University, Al Ain, 15551, UAE
4 Department of Humanities and Social Science, Al Ain University, Al Ain, 15551, UAE
5 Department of Computer Engineering, Korea Polytechnic University, Siheung-si, 237, Gyeonggi-do, Korea
* Corresponding Author: Jeongmin Park. Email:
Computers, Materials & Continua 2022, 72(3), 5327-5343. https://doi.org/10.32604/cmc.2022.025953
Received 09 December 2021; Accepted 04 March 2022; Issue published 21 April 2022
Abstract
In this research work, an efficient sign language recognition tool for e-learning has been proposed with a new type of feature set based on angle and lines. This feature set has the ability to increase the overall performance of machine learning algorithms in an efficient way. The hand gesture recognition based on these features has been implemented for usage in real-time. The feature set used hand landmarks, which were generated using media-pipe (MediaPipe) and open computer vision (openCV) on each frame of the incoming video. The overall algorithm has been tested on two well-known ASL-alphabet (American Sign Language) and ISL-HS (Irish Sign Language) sign language datasets. Different machine learning classifiers including random forest, decision tree, and naïve Bayesian have been used to classify hand gestures using this unique feature set and their respective results have been compared. Since the random forest classifier performed better, it has been selected as the base classifier for the proposed system. It showed 96.7% accuracy with ISL-HS and 93.7% accuracy with ASL-alphabet dataset using the extracted features.Keywords
Cite This Article
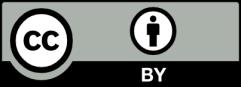