Open Access
ARTICLE
3D Instance Segmentation Using Deep Learning on RGB-D Indoor Data
1 Department of Robot System Engineering, Tongmyong University, Busan, 48520, Korea
2 Department of Information & Technology, University of Central Punjab, Lahore, Pakistan
3 Department of Electronics Engineering, Tongmyong University, Busan, 48520, Korea
* Corresponding Author: Hyunsik Ahn. Email:
Computers, Materials & Continua 2022, 72(3), 5777-5791. https://doi.org/10.32604/cmc.2022.025909
Received 08 December 2021; Accepted 02 March 2022; Issue published 21 April 2022
Abstract
3D object recognition is a challenging task for intelligent and robot systems in industrial and home indoor environments. It is critical for such systems to recognize and segment the 3D object instances that they encounter on a frequent basis. The computer vision, graphics, and machine learning fields have all given it a lot of attention. Traditionally, 3D segmentation was done with hand-crafted features and designed approaches that didn’t achieve acceptable performance and couldn’t be generalized to large-scale data. Deep learning approaches have lately become the preferred method for 3D segmentation challenges by their great success in 2D computer vision. However, the task of instance segmentation is currently less explored. In this paper, we propose a novel approach for efficient 3D instance segmentation using red green blue and depth (RGB-D) data based on deep learning. The 2D region based convolutional neural networks (Mask R-CNN) deep learning model with point based rending module is adapted to integrate with depth information to recognize and segment 3D instances of objects. In order to generate 3D point cloud coordinates (x, y, z), segmented 2D pixels (u, v) of recognized object regions in the RGB image are merged into (u, v) points of the depth image. Moreover, we conducted an experiment and analysis to compare our proposed method from various points of view and distances. The experimentation shows the proposed 3D object recognition and instance segmentation are sufficiently beneficial to support object handling in robotic and intelligent systems.Keywords
Cite This Article
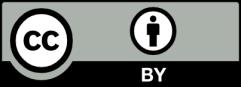
This work is licensed under a Creative Commons Attribution 4.0 International License , which permits unrestricted use, distribution, and reproduction in any medium, provided the original work is properly cited.