Open Access
ARTICLE
Non-Invasive Early Diagnosis of Obstructive Lung Diseases Leveraging Machine Learning Algorithms
1 Department of Electrical Engineering, Riphah International University, Islamabad, 44000, Pakistan
2 Security Engineering Lab, Prince Sultan University, Riyadh, 12435, Saudi Arabia
3 RIADI Laboratory, National School of Computer Science, University of Manouba, Manouba, 2010, Tunisia
4 Peoples’ Friendship University of Russia (RUDN University), Moscow, 117198, Russia
* Corresponding Author: Mujeeb Ur Rehman. Email:
Computers, Materials & Continua 2022, 72(3), 5681-5697. https://doi.org/10.32604/cmc.2022.025840
Received 07 December 2021; Accepted 18 March 2022; Issue published 21 April 2022
Abstract
Lungs are a vital human body organ, and different Obstructive Lung Diseases (OLD) such as asthma, bronchitis, or lung cancer are caused by shortcomings within the lungs. Therefore, early diagnosis of OLD is crucial for such patients suffering from OLD since, after early diagnosis, breathing exercises and medical precautions can effectively improve their health state. A secure non-invasive early diagnosis of OLD is a primordial need, and in this context, digital image processing supported by Artificial Intelligence (AI) techniques is reliable and widely used in the medical field, especially for improving early disease diagnosis. Hence, this article presents an AI-based non-invasive and secured diagnosis for OLD using physiological and iris features. This research work implements different machine-learning-based techniques which classify various subjects, which are healthy and effective patients. The iris features include gray-level run-length matrix-based features, gray-level co-occurrence matrix, and statistical features. These features are extracted from iris images. Additionally, ten different classifiers and voting techniques, including hard and soft voting, are implemented and tested, and their performances are evaluated using several parameters, which are precision, accuracy, specificity, F-score, and sensitivity. Based on the statistical analysis, it is concluded that the proposed approach offers promising techniques for the non-invasive early diagnosis of OLD with an accuracy of 97.6%.Keywords
Cite This Article
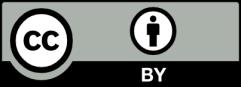