Open Access
ARTICLE
A Novel Convolutional Neural Network Model for Malaria Cell Images Classification
1 Faculty of Artificial Intelligence, Kafrelsheikh University, Kafrelsheikh, 33511, Egypt
2 Department of Information Technology, Faculty of Computers and Information, Mansoura University, Mansoura, 35516, Egypt
3 Department of Computer Science, Faculty of Computers and Information, Mansoura University, Mansoura, 35516, Egypt
* Corresponding Author: Esraa Hassan. Email:
Computers, Materials & Continua 2022, 72(3), 5889-5907. https://doi.org/10.32604/cmc.2022.025629
Received 30 November 2021; Accepted 23 March 2022; Issue published 21 April 2022
Abstract
Infectious diseases are an imminent danger that faces human beings around the world. Malaria is considered a highly contagious disease. The diagnosis of various diseases, including malaria, was performed manually, but it required a lot of time and had some human errors. Therefore, there is a need to investigate an efficient and fast automatic diagnosis system. Deploying deep learning algorithms can provide a solution in which they can learn complex image patterns and have a rapid improvement in medical image analysis. This study proposed a Convolutional Neural Network (CNN) model to detect malaria automatically. A Malaria Convolutional Neural Network (MCNN) model is proposed in this work to classify the infected cases. MCNN focuses on detecting infected cells, which aids in the computation of parasitemia, or infection measures. The proposed model achieved 0.9929, 0.9848, 0.9859, 0.9924, 0.0152, 0.0141, 0.0071, 0.9890, 0.9894, and 0.9780 in terms of specificity, sensitivity, precision, accuracy, F1-score, and Matthews Correlation Coefficient, respectively. A comparison was carried out between the proposed model and some recent works in the literature. This comparison demonstrates that the proposed model outperforms the compared works in terms of evaluation metrics.Keywords
Cite This Article
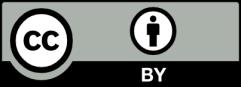
This work is licensed under a Creative Commons Attribution 4.0 International License , which permits unrestricted use, distribution, and reproduction in any medium, provided the original work is properly cited.