Open Access
ARTICLE
Arabic Music Genre Classification Using Deep Convolutional Neural Networks (CNNs)
1 Department of Software Engineering, Al-Hussein Bin Talal University, Ma’an, 71111, Jordan
2 Department of Computer Science, Al-Hussein Bin Talal University, Ma’an, 71111, Jordan
3 King Abdullah II School of Information Technology, The University of Jordan, Amman, Jordan
4 Department of Computer Science and Engineering, University of Bridgeport, Bridgeport, CT, 06604, USA
* Corresponding Author: Laiali Almazaydeh. Email: laiali.almazaydeh@ ahu.edu.jo
Computers, Materials & Continua 2022, 72(3), 5443-5458. https://doi.org/10.32604/cmc.2022.025526
Received 26 November 2021; Accepted 02 March 2022; Issue published 21 April 2022
Abstract
Genres are one of the key features that categorize music based on specific series of patterns. However, the Arabic music content on the web is poorly defined into its genres, making the automatic classification of Arabic audio genres challenging. For this reason, in this research, our objective is first to construct a well-annotated dataset of five of the most well-known Arabic music genres, which are: Eastern Takht, Rai, Muwashshah, the poem, and Mawwal, and finally present a comprehensive empirical comparison of deep Convolutional Neural Networks (CNNs) architectures on Arabic music genres classification. In this work, to utilize CNNs to develop a practical classification system, the audio data is transformed into a visual representation (spectrogram) using Short Time Fast Fourier Transformation (STFT), then several audio features are extracted using Mel Frequency Cepstral Coefficients (MFCC). Performance evaluation of classifiers is measured with the accuracy score, time to build, and Matthew’s correlation coefficient (MCC). The concluded results demonstrated that AlexNet is considered among the top-performing five CNNs classifiers studied: LeNet5, AlexNet, VGG, ResNet-50, and LSTM-CNN, with an overall accuracy of 96%.Keywords
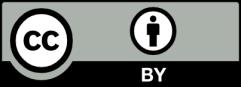