Open Access
ARTICLE
A Novel Deep Learning Based Healthcare Model for COVID-19 Pandemic Stress Analysis
1 Computer Science and Engineering, Women Institute of Technology, Uttarakhand-248007, India
2 Chitkara University Institute of Engineering and Technology, Chitkara University, Punjab, India
3 Research and Innovation, Uttranchal University, Dehradun, Uttrakhand, 248007, India
4 Department of Computer Science & IT, MJP Rohilkhand University, Bareilly, 236006, UP, India
5 Computer Science and Engineering, Lovely Professional University, Punjab, 144411, India
6 Higher Polytechnic School, Universidad Europea del Atlántico, 39011, Santander, Spain
7 Faculty of Computing and Information Technology, King Abdulaziz University, 37848, Jeddah, Saudi Arabia
8 Universidad Internacional Iberoamericana, Campeche, Mexico
9 Universidade Internacional do Cuanza Bairro Kaluanda, EN 250, Cuito, Bié. Angola
* Corresponding Author: Divya Anand. Email:
Computers, Materials & Continua 2022, 72(3), 6029-6044. https://doi.org/10.32604/cmc.2022.024698
Received 28 October 2021; Accepted 14 January 2022; Issue published 21 April 2022
Abstract
Coronavirus (COVID-19) has impacted nearly every person across the globe either in terms of losses of life or as of lockdown. The current coronavirus (COVID-19) pandemic is a rare/special situation where people can express their feelings on Internet-based social networks. Social media is emerging as the biggest platform in recent years where people spend most of their time expressing themselves and their emotions. This research is based on gathering data from Twitter and analyzing the behavior of the people during the COVID-19 lockdown. The research is based on the logic expressed by people in this perspective and emotions for the suffering of COVID-19 and lockdown. In this research, we have used a Long Short-Term Memory (LSTM) network model with Convolutional Neural Network using Keras python deep-learning library to determine whether social media platform users are depressed in terms of positive, negative, or neutral emotional out bust based on their Twitter posts. The results showed that the model has 88.14% accuracy (representation of the correct prediction over the test dataset) after 10 epochs which most tweets showed had neutral polarity. The evaluation shows interesting results in positive (1), negative (–1), and neutral (0) emotions through different visualization.Keywords
Cite This Article
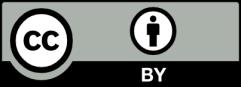
This work is licensed under a Creative Commons Attribution 4.0 International License , which permits unrestricted use, distribution, and reproduction in any medium, provided the original work is properly cited.