Open Access
ARTICLE
Brain Tumor Segmentation using Multi-View Attention based Ensemble Network
1 COMSATS University Islamabad, Islamabad Campus, 45550, Pakistan
2 Suranaree University of Technology, Nakhon Ratchasima, 30000, Thailand
3 COMSATS University Islamabad, Lahore Campus, 54000, Pakistan
4 Virtual University of Pakistan, Islamabad Campus, 45550, Pakistan
5 COMSATS University Islamabad, Vehari Campus, 61100, Pakistan
* Corresponding Author: Chitapong Wechtaisong. Email:
Computers, Materials & Continua 2022, 72(3), 5793-5806. https://doi.org/10.32604/cmc.2022.024316
Received 13 October 2021; Accepted 02 December 2021; Issue published 21 April 2022
Abstract
Astrocytoma IV or glioblastoma is one of the fatal and dangerous types of brain tumors. Early detection of brain tumor increases the survival rate and helps in reducing the fatality rate. Various imaging modalities have been used for diagnosing by expert radiologists, and Medical Resonance Image (MRI) is considered a better option for detecting brain tumors as MRI is a non-invasive technique and provides better visualization of the brain region. One of the challenging issues is to identify the tumorous region from the MRI scans correctly. Manual segmentation is performed by medical experts, which is a time-consuming task and got chances of errors. To overcome this issue, automatic segmentation is performed for quick and accurate results. The proposed approach is to capture inter-slice information and reduce the outliers. Deep learning-based brain tumor segmentation techniques proved best among available segmentation techniques. However, deep learning may miss some preliminary info while using MRI images during segmentation. As MRI volumes are volumetric, 3D U-Net-based models are used but complex. Combinations of multiple 2D U-Net predictions in axial, sagittal, and coronal views help to capture inter-slice information. This approach may reduce the system complexity. Moreover, the Conditional Random Fields (CRF) reduce the predictions’ false positives and improve the segmentation results. This model is applied to Brain Tumor Segmentation (BraTS) 2019 dataset, and cross-validation is performed to check the accuracy of results. The proposed approach achieves Dice Similarity Score (DSC) of 0.77 on Enhancing Tumor (ET), 0.90 on Whole Tumor (WT), and 0.84 on Tumor Core (TC) with reduced Hausdorff Distance (HD) of 3.05 on ET, 5.12 on WT and 3.89 on TC.Keywords
Cite This Article
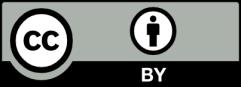
This work is licensed under a Creative Commons Attribution 4.0 International License , which permits unrestricted use, distribution, and reproduction in any medium, provided the original work is properly cited.