Open Access
ARTICLE
Multi-Stream CNN-Based Personal Recognition Method Using Surface Electromyogram for 5G Security
1 IT Research Institute, Chosun University, Gwangju, 61452, Korea
2 Interdisciplinary Program in IT-Bio Convergence System, Chosun University, Gwangju, 61452, Korea
* Corresponding Author: Sung Bum Pan. Email:
Computers, Materials & Continua 2022, 72(2), 2997-3007. https://doi.org/10.32604/cmc.2022.026572
Received 30 December 2021; Accepted 07 February 2022; Issue published 29 March 2022
Abstract
As fifth generation technology standard (5G) technology develops, the possibility of being exposed to the risk of cyber-attacks that exploits vulnerabilities in the 5G environment is increasing. The existing personal recognition method used for granting permission is a password-based method, which causes security problems. Therefore, personal recognition studies using bio-signals are being conducted as a method to access control to devices. Among bio-signal, surface electromyogram (sEMG) can solve the existing personal recognition problem that was unable to the modification of registered information owing to the characteristic changes in its signal according to the performed operation. Furthermore, as an advantage, sEMG can be conveniently measured from arms and legs. This paper proposes a personal recognition method using sEMG, based on a multi-stream convolutional neural network (CNN). The proposed method decomposes sEMG signals into intrinsic mode functions (IMF) using empirical mode decomposition (EMD) and transforms each IMF into a spectrogram. Personal recognition is performed by analyzing time–frequency features from the spectrogram transformed into multi-stream CNN. The database (DB) adopted in this paper is the Ninapro DB, which is a benchmark EMG DB. The experimental results indicate that the personal recognition performance of the multi-stream CNN using the IMF spectrogram improved by 1.91%, compared with the single-stream CNN using the spectrogram of raw sEMG.Keywords
Cite This Article
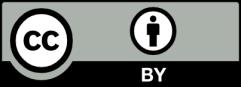
This work is licensed under a Creative Commons Attribution 4.0 International License , which permits unrestricted use, distribution, and reproduction in any medium, provided the original work is properly cited.