Open Access
ARTICLE
Automated Handwriting Recognition and Speech Synthesizer for Indigenous Language Processing
1 MIS Department, College of Business Administration, University of Business and Technology, Jeddah, 21448, Saudi Arabia
2 Department of Computer Science, University College of Duba, University of Tabuk, 71491, Saudi Arabia
3 School of Engineering, Princess Sumaya University for Technology, Amman, 11941, Jordan
* Corresponding Author: Bassam A. Y. Alqaralleh. Email:
Computers, Materials & Continua 2022, 72(2), 3913-3927. https://doi.org/10.32604/cmc.2022.026531
Received 29 December 2021; Accepted 23 February 2022; Issue published 29 March 2022
Abstract
In recent years, researchers in handwriting recognition analysis relating to indigenous languages have gained significant internet among research communities. The recent developments of artificial intelligence (AI), natural language processing (NLP), and computational linguistics (CL) find useful in the analysis of regional low resource languages. Automatic lexical task participation might be elaborated to various applications in the NLP. It is apparent from the availability of effective machine recognition models and open access handwritten databases. Arabic language is a commonly spoken Semitic language, and it is written with the cursive Arabic alphabet from right to left. Arabic handwritten Character Recognition (HCR) is a crucial process in optical character recognition. In this view, this paper presents effective Computational linguistics with Deep Learning based Handwriting Recognition and Speech Synthesizer (CLDL-THRSS) for Indigenous Language. The presented CLDL-THRSS model involves two stages of operations namely automated handwriting recognition and speech recognition. Firstly, the automated handwriting recognition procedure involves preprocessing, segmentation, feature extraction, and classification. Also, the Capsule Network (CapsNet) based feature extractor is employed for the recognition of handwritten Arabic characters. For optimal hyperparameter tuning, the cuckoo search (CS) optimization technique was included to tune the parameters of the CapsNet method. Besides, deep neural network with hidden Markov model (DNN-HMM) model is employed for the automatic speech synthesizer. To validate the effective performance of the proposed CLDL-THRSS model, a detailed experimental validation process takes place and investigates the outcomes interms of different measures. The experimental outcomes denoted that the CLDL-THRSS technique has demonstrated the compared methods.Keywords
Cite This Article
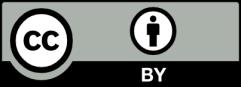