Open Access
ARTICLE
An Interpretable Artificial Intelligence Based Smart Agriculture System
1 School of Engineering and Technology, Central Queensland University, Sydney, Australia
2 College of Engineering, Science and Environment, The University of Newcastle, Sydney, Australia
3 School of Social Sciences, Western Sydney University, Sydney, Australia
4 Department of Environmental Sciences, Macquarie University, Sydney, Australia
5 School of Computing, Data and Mathematical, Sciences, Western Sydney University, Sydney, Australia
6 School of Engineering and Technology, Central Queensland University, Cairns, Australia
* Corresponding Author: Fariza Sabrina. Email:
Computers, Materials & Continua 2022, 72(2), 3777-3797. https://doi.org/10.32604/cmc.2022.026363
Received 23 December 2021; Accepted 22 February 2022; Issue published 29 March 2022
Abstract
With increasing world population the demand of food production has increased exponentially. Internet of Things (IoT) based smart agriculture system can play a vital role in optimising crop yield by managing crop requirements in real-time. Interpretability can be an important factor to make such systems trusted and easily adopted by farmers. In this paper, we propose a novel artificial intelligence-based agriculture system that uses IoT data to monitor the environment and alerts farmers to take the required actions for maintaining ideal conditions for crop production. The strength of the proposed system is in its interpretability which makes it easy for farmers to understand, trust and use it. The use of fuzzy logic makes the system customisable in terms of types/number of sensors, type of crop, and adaptable for any soil types and weather conditions. The proposed system can identify anomalous data due to security breaches or hardware malfunction using machine learning algorithms. To ensure the viability of the system we have conducted thorough research related to agricultural factors such as soil type, soil moisture, soil temperature, plant life cycle, irrigation requirement and water application timing for Maize as our target crop. The experimental results show that our proposed system is interpretable, can detect anomalous data, and triggers actions accurately based on crop requirements.Keywords
Cite This Article
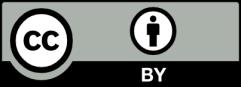