Open Access
ARTICLE
Metaheuristics with Deep Learning Empowered Biomedical Atherosclerosis Disease Diagnosis and Classification
1 Department of Industrial and Systems Engineering, College of Engineering, Princess Nourah Bint Abdulrahman University, Riyadh, 11671, Saudi Arabia
2 Department of Computer Science, College of Science and Arts, King Khalid University, Mahayil, Asir, Saudi Arabia
3 Department of Computer and Self Development, Preparatory Year Deanship, Prince Sattam bin Abdulaziz University, Al-Kharj, 16278, Saudi Arabia
* Corresponding Author: Manar Ahmed Hamza. Email:
Computers, Materials & Continua 2022, 72(2), 2859-2875. https://doi.org/10.32604/cmc.2022.026338
Received 22 December 2021; Accepted 24 January 2022; Issue published 29 March 2022
Abstract
Atherosclerosis diagnosis is an inarticulate and complicated cognitive process. Researches on medical diagnosis necessitate maximum accuracy and performance to make optimal clinical decisions. Since the medical diagnostic outcomes need to be prompt and accurate, the recently developed artificial intelligence (AI) and deep learning (DL) models have received considerable attention among research communities. This study develops a novel Metaheuristics with Deep Learning Empowered Biomedical Atherosclerosis Disease Diagnosis and Classification (MDL-BADDC) model. The proposed MDL-BADDC technique encompasses several stages of operations such as pre-processing, feature selection, classification, and parameter tuning. Besides, the proposed MDL-BADDC technique designs a novel Quasi-Oppositional Barnacles Mating Optimizer (QOBMO) based feature selection technique. Moreover, the deep stacked autoencoder (DSAE) based classification model is designed for the detection and classification of atherosclerosis disease. Furthermore, the krill herd algorithm (KHA) based parameter tuning technique is applied to properly adjust the parameter values. In order to showcase the enhanced classification performance of the MDL-BADDC technique, a wide range of simulations take place on three benchmarks biomedical datasets. The comparative result analysis reported the better performance of the MDL-BADDC technique over the compared methods.Keywords
Cite This Article
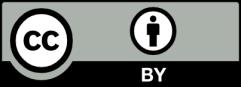
This work is licensed under a Creative Commons Attribution 4.0 International License , which permits unrestricted use, distribution, and reproduction in any medium, provided the original work is properly cited.