Open Access
ARTICLE
Intelligent Feature Selection with Deep Learning Based Financial Risk Assessment Model
1 College of Computer Engineering and Sciences, Prince Sattam bin Abdulaziz University, Al-Kharj, 16278, Saudi Arabia
2 Department of Electronics and Communication Engineering, K.Ramakrishnan College of Engineering, Tiruchirappalli, 621112, India
3 Department of Computer Science and Engineering, Panimalar Engineering College, Chennai, 600 123, India
4 Department of Electronics and Communication Engineering, M. Kumarasamy College of Engineering, Karur, 639113, India
5 Department of Computer Science and Information System, College of Applied Sciences, AlMaarefa University, Riyadh, 11597, Kingdom of Saudi Arabia
6 Department of Entrepreneurship and Logistics, Plekhanov Russian University of Economics, 117997, Moscow, Russia
7 Department of Logistics, State University of Management, 109542, Moscow, Russia
* Corresponding Author: Irina V. Pustokhina. Email:
Computers, Materials & Continua 2022, 72(2), 2429-2444. https://doi.org/10.32604/cmc.2022.026204
Received 18 December 2021; Accepted 24 January 2022; Issue published 29 March 2022
Abstract
Due to global financial crisis, risk management has received significant attention to avoid loss and maximize profit in any business. Since the financial crisis prediction (FCP) process is mainly based on data driven decision making and intelligent models, artificial intelligence (AI) and machine learning (ML) models are widely utilized. This article introduces an intelligent feature selection with deep learning based financial risk assessment model (IFSDL-FRA). The proposed IFSDL-FRA technique aims to determine the financial crisis of a company or enterprise. In addition, the IFSDL-FRA technique involves the design of new water strider optimization algorithm based feature selection (WSOA-FS) manner to an optimum selection of feature subsets. Moreover, Deep Random Vector Functional Link network (DRVFLN) classification technique was applied to properly allot the class labels to the financial data. Furthermore, improved fruit fly optimization algorithm (IFFOA) based hyperparameter tuning process is carried out to optimally tune the hyperparameters of the DRVFLN model. For enhancing the better performance of the IFSDL-FRA technique, an extensive set of simulations are implemented on benchmark financial datasets and the obtained outcomes determine the betterment of IFSDL-FRA technique on the recent state of art approaches.Keywords
Cite This Article
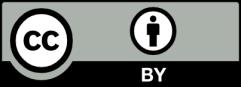