Open Access
ARTICLE
Heart Disease Diagnosis Using the Brute Force Algorithm and Machine Learning Techniques
1 Department of Computer Science and Engineering, Kongju National University, Cheonan, 31080, Korea
2 Department of Computer Science, COMSATS University Islamabad, Wah Campus, 47040, Pakistan
3 School of Computer Science, University of Sydney, Sydney, NSW 2006, Australia
4 Centre of AI and Data Science, Edinburgh Napier University, Edinburgh, EH11 4DY, UK
* Corresponding Author: Jungeun Kim. Email:
Computers, Materials & Continua 2022, 72(2), 3195-3211. https://doi.org/10.32604/cmc.2022.026064
Received 14 December 2021; Accepted 11 February 2022; Issue published 29 March 2022
Abstract
Heart disease is one of the leading causes of death in the world today. Prediction of heart disease is a prominent topic in the clinical data processing. To increase patient survival rates, early diagnosis of heart disease is an important field of research in the medical field. There are many studies on the prediction of heart disease, but limited work is done on the selection of features. The selection of features is one of the best techniques for the diagnosis of heart diseases. In this research paper, we find optimal features using the brute-force algorithm, and machine learning techniques are used to improve the accuracy of heart disease prediction. For performance evaluation, accuracy, sensitivity, and specificity are used with split and cross-validation techniques. The results of the proposed technique are evaluated in three different heart disease datasets with a different number of records, and the proposed technique is found to have superior performance. The selection of optimized features generated by the brute force algorithm is used as input to machine learning algorithms such as Support Vector Machine (SVM), Random Forest (RF), K Nearest Neighbor (KNN), and Naive Bayes (NB). The proposed technique achieved 97% accuracy with Naive Bayes through split validation and 95% accuracy with Random Forest through cross-validation. Naive Bayes and Random Forest are found to outperform other classification approaches when accurately evaluated. The results of the proposed technique are compared with the results of the existing study, and the results of the proposed technique are found to be better than other state-of-the-art methods. Therefore, our proposed approach plays an important role in the selection of important features and the automatic detection of heart disease.Keywords
Cite This Article
Citations
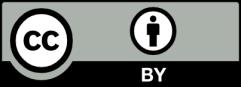
This work is licensed under a Creative Commons Attribution 4.0 International License , which permits unrestricted use, distribution, and reproduction in any medium, provided the original work is properly cited.