Open Access
ARTICLE
Effective Classification of Synovial Sarcoma Cancer Using Structure Features and Support Vectors
1 Department of Electronics and Communication Engineering, K.L.N. College of Engineering, Pottapalayam, 630612, Tamil Nadu, India
2 Department of Computer Science and Engineering, Kongju National University, Cheonan 31080, Korea
3 Department of Mathematics, Tamralipta Mahavidyalaya, Tamluk, 721636, West Bengal
4 School of Computer Science, University of Sydney, NSW 2006, Australia
5 School of Computer Science and Engineering, Vellore Institute of Technology, Chennai, 600127, India
6 School of Computer Science and Engineering, Centre for Cyber Physical Systems, Vellore Institute of Technology, Chennai, 600127, India
* Corresponding Author: Jungeun Kim. Email:
Computers, Materials & Continua 2022, 72(2), 2521-2543. https://doi.org/10.32604/cmc.2022.025339
Received 20 November 2021; Accepted 06 January 2022; Issue published 29 March 2022
Abstract
In this research work, we proposed a medical image analysis framework with two separate releases whether or not Synovial Sarcoma (SS) is the cell structure for cancer. Within this framework the histopathology images are decomposed into a third-level sub-band using a two-dimensional Discrete Wavelet Transform. Subsequently, the structure features (SFs) such as Principal Components Analysis (PCA), Independent Components Analysis (ICA) and Linear Discriminant Analysis (LDA) were extracted from this sub-band image representation with the distribution of wavelet coefficients. These SFs are used as inputs of the Support Vector Machine (SVM) classifier. Also, classification of PCA + SVM, ICA + SVM, and LDA + SVM with Radial Basis Function (RBF) kernel the efficiency of the process is differentiated and compared with the best classification results. Furthermore, data collected on the internet from various histopathological centres via the Internet of Things (IoT) are stored and shared on blockchain technology across a wide range of image distribution across secure data IoT devices. Due to this, the minimum and maximum values of the kernel parameter are adjusted and updated periodically for the purpose of industrial application in device calibration. Consequently, these resolutions are presented with an excellent example of a technique for training and testing the cancer cell structure prognosis methods in spindle shaped cell (SSC) histopathological imaging databases. The performance characteristics of cross-validation are evaluated with the help of the receiver operating characteristics (ROC) curve, and significant differences in classification performance between the techniques are analyzed. The combination of LDA + SVM technique has been proven to be essential for intelligent SS cancer detection in the future, and it offers excellent classification accuracy, sensitivity, specificity.Keywords
Cite This Article
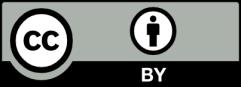
This work is licensed under a Creative Commons Attribution 4.0 International License , which permits unrestricted use, distribution, and reproduction in any medium, provided the original work is properly cited.