Open Access
ARTICLE
Improving Association Rules Accuracy in Noisy Domains Using Instance Reduction Techniques
1 College of Computing and Informatics, Saudi Electronic University, Riyadh, 11673, Saudi Arabia
2 King Abdullah II School for Information Technology, The University of Jordan, Amman, 11942, Jordan
3 Faculty of Engineering, Port Said University, Port Said, 42523, Egypt
* Corresponding Author: Mousa Al-Akhras. Email:
Computers, Materials & Continua 2022, 72(2), 3719-3749. https://doi.org/10.32604/cmc.2022.025196
Received 16 November 2021; Accepted 18 February 2022; Issue published 29 March 2022
Abstract
Association rules’ learning is a machine learning method used in finding underlying associations in large datasets. Whether intentionally or unintentionally present, noise in training instances causes overfitting while building the classifier and negatively impacts classification accuracy. This paper uses instance reduction techniques for the datasets before mining the association rules and building the classifier. Instance reduction techniques were originally developed to reduce memory requirements in instance-based learning. This paper utilizes them to remove noise from the dataset before training the association rules classifier. Extensive experiments were conducted to assess the accuracy of association rules with different instance reduction techniques, namely: Decremental Reduction Optimization Procedure (DROP) 3, DROP5, ALL K-Nearest Neighbors (ALLKNN), Edited Nearest Neighbor (ENN), and Repeated Edited Nearest Neighbor (RENN) in different noise ratios. Experiments show that instance reduction techniques substantially improved the average classification accuracy on three different noise levels: 0%, 5%, and 10%. The RENN algorithm achieved the highest levels of accuracy with a significant improvement on seven out of eight used datasets from the University of California Irvine (UCI) machine learning repository. The improvements were more apparent in the 5% and the 10% noise cases. When RENN was applied, the average classification accuracy for the eight datasets in the zero-noise test enhanced from 70.47% to 76.65% compared to the original test. The average accuracy was improved from 66.08% to 77.47% for the 5%-noise case and from 59.89% to 77.59% in the 10%-noise case. Higher confidence was also reported in building the association rules when RENN was used. The above results indicate that RENN is a good solution in removing noise and avoiding overfitting during the construction of the association rules classifier, especially in noisy domains.Keywords
Cite This Article
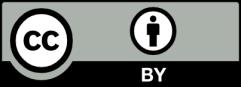
This work is licensed under a Creative Commons Attribution 4.0 International License , which permits unrestricted use, distribution, and reproduction in any medium, provided the original work is properly cited.