Open Access
ARTICLE
Detection of Lung Tumor Using ASPP-Unet with Whale Optimization Algorithm
1 Department of Computer Science, College of Computer and Information Sciences, Prince Sultan University, 11564,Saudi Arabia
2 Department of Computer Science, College of Science and Arts, King Khalid University, Mahayil, Asir, 61913, Saudi Arabia
3 Department of Biomedical Engineering, College of Engineering, Princess Nourah Bint Abdulrahman University, Riyadh, 11671, Saudi Arabia
4 Department of Computer and Self Development, Preparatory Year Deanship, Prince Sattam bin Abdulaziz University, AlKharj, 16278, Saudi Arabia
5 Department of Natural and Applied Sciences, College of Community-Aflaj, Prince Sattam bin Abdulaziz University, 16278, Saudi Arabia
* Corresponding Author: Anwer Mustafa Hilal. Email:
Computers, Materials & Continua 2022, 72(2), 3511-3527. https://doi.org/10.32604/cmc.2022.024583
Received 23 October 2021; Accepted 17 January 2022; Issue published 29 March 2022
Abstract
The unstructured growth of abnormal cells in the lung tissue creates tumor. The early detection of lung tumor helps the patients avoiding the death rate and gives better treatment. Various medical image modalities can help the physicians in the diagnosis of disease. Many research works have been proposed for the early detection of lung tumor. High computation time and misidentification of tumor are the prevailing issues. In order to overcome these issues, this paper has proposed a hybrid classifier of Atrous Spatial Pyramid Pooling (ASPP)-Unet architecture with Whale Optimization Algorithm (ASPP-Unet -WOA). To get a fine tuning detection of tumor in the Computed Tomography (CT) of lung image, this model needs pre-processing using Gabor filter. Secondly, feature segmentation is done using Guaranteed Convergence Particle Swarm Optimization. Thirdly, feature selection is done using Binary Grasshopper Optimization Algorithm. This proposed (ASPP-Unet -WOA) is implemented in the dataset of National Cancer Institute (NCI) Lung Cancer Database Consortium. Various performance metric measures are evaluated and compared to the existing classifiers. The accuracy of Deep Convolutional Neural Network (DCNN) is 93.45%, Convolutional Neural Network (CNN) is 91.67%, UNet obtains 95.75% and ASPP-UNet-WOA obtains 98.68%. compared to the other techniques.Keywords
Cite This Article
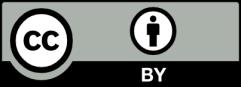
This work is licensed under a Creative Commons Attribution 4.0 International License , which permits unrestricted use, distribution, and reproduction in any medium, provided the original work is properly cited.