Open Access
ARTICLE
Hybrid Machine Learning Model for Face Recognition Using SVM
1 IES College of Technology, Bhopal, 462044, India
2 Maulana Azad National Institute of Technology, Bhopal, 462003, India
3 Department of Information Technology, Manipal University Jaipur, Jaipur, 303007, India
4 Department of Computer and Communication Engineering, Manipal University Jaipur, Jaipur, 303007, India
5 Department of Software Engineering, College of Computer Science and Engineering, University of Jeddah, Jeddah, 23218, Saudi Arabia
* Corresponding Author: Punit Gupta. Email:
Computers, Materials & Continua 2022, 72(2), 2697-2712. https://doi.org/10.32604/cmc.2022.023052
Received 26 August 2021; Accepted 28 December 2021; Issue published 29 March 2022
Abstract
Face recognition systems have enhanced human-computer interactions in the last ten years. However, the literature reveals that current techniques used for identifying or verifying faces are not immune to limitations. Principal Component Analysis-Support Vector Machine (PCA-SVM) and Principal Component Analysis-Artificial Neural Network (PCA-ANN) are among the relatively recent and powerful face analysis techniques. Compared to PCA-ANN, PCA-SVM has demonstrated generalization capabilities in many tasks, including the ability to recognize objects with small or large data samples. Apart from requiring a minimal number of parameters in face detection, PCA-SVM minimizes generalization errors and avoids overfitting problems better than PCA-ANN. PCA-SVM, however, is ineffective and inefficient in detecting human faces in cases in which there is poor lighting, long hair, or items covering the subject's face. This study proposes a novel PCA-SVM-based model to overcome the recognition problem of PCA-ANN and enhance face detection. The experimental results indicate that the proposed model provides a better face recognition outcome than PCA-SVM.Keywords
Cite This Article
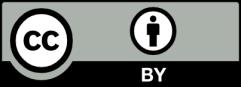
This work is licensed under a Creative Commons Attribution 4.0 International License , which permits unrestricted use, distribution, and reproduction in any medium, provided the original work is properly cited.