Open Access
ARTICLE
A Deep Learning Hierarchical Ensemble for Remote Sensing Image Classification
1 Department of Computer Engineering, Anyang University, Anyang-si, 14028, Korea
2 Department of ICT Convergence Engineering, Anyang University, Anyang-si, 14028, Korea
* Corresponding Author: Jeong-Joon Kim. Email:
Computers, Materials & Continua 2022, 72(2), 2649-2663. https://doi.org/10.32604/cmc.2022.022593
Received 12 August 2021; Accepted 13 September 2021; Issue published 29 March 2022
Abstract
Artificial intelligence, which has recently emerged with the rapid development of information technology, is drawing attention as a tool for solving various problems demanded by society and industry. In particular, convolutional neural networks (CNNs), a type of deep learning technology, are highlighted in computer vision fields, such as image classification and recognition and object tracking. Training these CNN models requires a large amount of data, and a lack of data can lead to performance degradation problems due to overfitting. As CNN architecture development and optimization studies become active, ensemble techniques have emerged to perform image classification by combining features extracted from multiple CNN models. In this study, data augmentation and contour image extraction were performed to overcome the data shortage problem. In addition, we propose a hierarchical ensemble technique to achieve high image classification accuracy, even if trained from a small amount of data. First, we trained the UC-Merced land use dataset and the contour images for each image on pretrained VGGNet, GoogLeNet, ResNet, DenseNet, and EfficientNet. We then apply a hierarchical ensemble technique to the number of cases in which each model can be deployed. These experiments were performed in cases where the proportion of training datasets was 30%, 50%, and 70%, resulting in a performance improvement of up to 4.68% compared to the average accuracy of the entire model.Keywords
Cite This Article
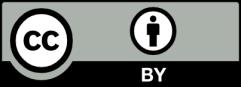
This work is licensed under a Creative Commons Attribution 4.0 International License , which permits unrestricted use, distribution, and reproduction in any medium, provided the original work is properly cited.