Open Access
ARTICLE
An Optimal Scheme for WSN Based on Compressed Sensing
1 Department of Information Systems and Networks, Faculty of Information Technology, The World Islamic Sciences & Education University, Jordan
2 Computer Science Department, Community College, King Saud University, Saudi Arabia
3 Department of Computer Engineering, National Technical University of Ukraine “Igor Sikorsky Kyiv Polytechnic Institute, Ukraine
* Corresponding Author: Ahmad Ali AlZubi. Email:
(This article belongs to the Special Issue: Big Data and Smart Cities Challenges)
Computers, Materials & Continua 2022, 72(1), 1053-1069. https://doi.org/10.32604/cmc.2022.025555
Received 28 November 2021; Accepted 28 December 2021; Issue published 24 February 2022
Abstract
Wireless sensor networks (WSNs) is one of the renowned ad hoc network technology that has vast varieties of applications such as in computer networks, bio-medical engineering, agriculture, industry and many more. It has been used in the internet-of-things (IoTs) applications. A method for data collecting utilizing hybrid compressive sensing (CS) is developed in order to reduce the quantity of data transmission in the clustered sensor network and balance the network load. Candidate cluster head nodes are chosen first from each temporary cluster that is closest to the cluster centroid of the nodes, and then the cluster heads are selected in order based on the distance between the determined cluster head node and the undetermined candidate cluster head node. Then, each ordinary node joins the cluster that is nearest to it. The greedy CS is used to compress data transmission for nodes whose data transmission volume is greater than the threshold in a data transmission tree with the Sink node as the root node and linking all cluster head nodes. The simulation results demonstrate that when the compression ratio is set to ten, the data transfer volume is reduced by a factor of ten. When compared to clustering and SPT without CS, it is reduced by 75% and 65%, respectively. When compared to SPT with Hybrid CS and Clustering with hybrid CS, it is reduced by 35% and 20%, respectively. Clustering and SPT without CS are compared in terms of node data transfer volume standard deviation. SPT with Hybrid CS and clustering with Hybrid CS were both reduced by 62% and 80%, respectively. When compared to SPT with hybrid CS and clustering with hybrid CS, the latter two were reduced by 41% and 19%, respectively.Keywords
Cite This Article
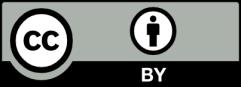
This work is licensed under a Creative Commons Attribution 4.0 International License , which permits unrestricted use, distribution, and reproduction in any medium, provided the original work is properly cited.