Open Access
ARTICLE
Intelligent Deep Data Analytics Based Remote Sensing Scene Classification Model
1 Department of Electrical Engineering, College of Engineering, Taif University, P.O. Box 11099, Taif, 21944, Saudi Arabia
2 Department of Science and Technology, College of Ranyah, Taif University, P.O. Box 11099, Taif, 21944, Saudi Arabia
3 Department of Mathematics, College of Science, P.O. Box 11099, Taif University, Taif, 21944, Saudi Arabia
4 Department of Computer Science, College of Computer, Qassim University, Buraydah, 51452, Saudi Arabia
5 Department of Mathematics, Faculty of Science, New Valley University, El-Kharga, 72511, Egypt
* Corresponding Author: Romany F. Mansour. Email:
Computers, Materials & Continua 2022, 72(1), 1921-1938. https://doi.org/10.32604/cmc.2022.025550
Received 27 November 2021; Accepted 11 January 2022; Issue published 24 February 2022
Abstract
Latest advancements in the integration of camera sensors paves a way for new Unmanned Aerial Vehicles (UAVs) applications such as analyzing geographical (spatial) variations of earth science in mitigating harmful environmental impacts and climate change. UAVs have achieved significant attention as a remote sensing environment, which captures high-resolution images from different scenes such as land, forest fire, flooding threats, road collision, landslides, and so on to enhance data analysis and decision making. Dynamic scene classification has attracted much attention in the examination of earth data captured by UAVs. This paper proposes a new multi-modal fusion based earth data classification (MMF-EDC) model. The MMF-EDC technique aims to identify the patterns that exist in the earth data and classifies them into appropriate class labels. The MMF-EDC technique involves a fusion of histogram of gradients (HOG), local binary patterns (LBP), and residual network (ResNet) models. This fusion process integrates many feature vectors and an entropy based fusion process is carried out to enhance the classification performance. In addition, the quantum artificial flora optimization (QAFO) algorithm is applied as a hyperparameter optimization technique. The AFO algorithm is inspired by the reproduction and the migration of flora helps to decide the optimal parameters of the ResNet model namely learning rate, number of hidden layers, and their number of neurons. Besides, Variational Autoencoder (VAE) based classification model is applied to assign appropriate class labels for a useful set of feature vectors. The proposed MMF-EDC model has been tested using UCM and WHU-RS datasets. The proposed MMF-EDC model attains exhibits promising classification results on the applied remote sensing images with the accuracy of 0.989 and 0.994 on the test UCM and WHU-RS dataset respectively.Keywords
Cite This Article
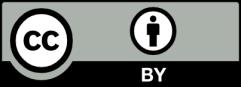