Open Access
ARTICLE
Energy Theft Identification Using Adaboost Ensembler in the Smart Grids
1 Electrical Engineering Department, College of Engineering, Najran University Saudi Arabia, Najran, 61441, Saudi Arabia
2 Department of Computer Science, Federal Urdu University of Science and Technology, Islamabad, 44000, Pakistan
3 Faculty of Maritime Studies, King Abdulaziz University, Jeddah, 21589, Saudi Arabia
4 Department of Electrical Engineering, HITEC University, Taxila, 47080, Pakistan
5 Department of Computer Science and Engineering, University of Engineering and Technology, Narowal Campus, Lahore, 54000, Pakistan
6 College of Computer Science and Information Systems, Najran University, Najran, 61441, Saudi Arabia
7 Department of Electrical and Power Engineering, AGH University of Science and Technology Mickiewicza 30 Avenue, Krakow, 30-059, Poland
* Corresponding Author: Muhammad Irfan. Email:
(This article belongs to the Special Issue: Towards Big Data Analytics: Smart and Intelligent Techniques for Sustainable Smart Grid)
Computers, Materials & Continua 2022, 72(1), 2141-2158. https://doi.org/10.32604/cmc.2022.025466
Received 24 November 2021; Accepted 07 January 2022; Issue published 24 February 2022
Abstract
One of the major concerns for the utilities in the Smart Grid (SG) is electricity theft. With the implementation of smart meters, the frequency of energy usage and data collection from smart homes has increased, which makes it possible for advanced data analysis that was not previously possible. For this purpose, we have taken historical data of energy thieves and normal users. To avoid imbalance observation, biased estimates, we applied the interpolation method. Furthermore, the data unbalancing issue is resolved in this paper by Nearmiss undersampling technique and makes the data suitable for further processing. By proposing an improved version of Zeiler and Fergus Net (ZFNet) as a feature extraction approach, we had able to reduce the model's time complexity. To minimize the overfitting issues, increase the training accuracy and reduce the training loss, we have proposed an enhanced method by merging Adaptive Boosting (AdaBoost) classifier with Coronavirus Herd Immunity Optimizer (CHIO) and Forensic based Investigation Optimizer (FBIO). In terms of low computational complexity, minimized over-fitting problems on a large quantity of data, reduced training time and training loss and increased training accuracy, our model outperforms the benchmark scheme. Our proposed algorithms Ada-CHIO and Ada-FBIO, have the low Mean Average Percentage Error (MAPE) value of error, i.e., 6.8% and 9.5%, respectively. Furthermore, due to the stability of our model our proposed algorithms Ada-CHIO and Ada-FBIO have achieved the accuracy of 93% and 90%. Statistical analysis shows that the hypothesis we proved using statistics is authentic for the proposed technique against benchmark algorithms, which also depicts the superiority of our proposed techniquesKeywords
Cite This Article
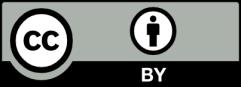
This work is licensed under a Creative Commons Attribution 4.0 International License , which permits unrestricted use, distribution, and reproduction in any medium, provided the original work is properly cited.