Open Access
ARTICLE
Embedding Extraction for Arabic Text Using the AraBERT Model
1 Faculty of Computers and Information, Department of Computer Sciences, Mansoura University, Mansoura,35516, Egypt
2 Faculty of Computers and Artificial Intelligence, Department of Computer Sciences, Damietta University, Damietta, 34517, Egypt
* Corresponding Author: Amira Hamed Abo-Elghit. Email:
Computers, Materials & Continua 2022, 72(1), 1967-1994. https://doi.org/10.32604/cmc.2022.025353
Received 21 November 2021; Accepted 17 January 2022; Issue published 24 February 2022
Abstract
Nowadays, we can use the multi-task learning approach to train a machine-learning algorithm to learn multiple related tasks instead of training it to solve a single task. In this work, we propose an algorithm for estimating textual similarity scores and then use these scores in multiple tasks such as text ranking, essay grading, and question answering systems. We used several vectorization schemes to represent the Arabic texts in the SemEval2017-task3-subtask-D dataset. The used schemes include lexical-based similarity features, frequency-based features, and pre-trained model-based features. Also, we used contextual-based embedding models such as Arabic Bidirectional Encoder Representations from Transformers (AraBERT). We used the AraBERT model in two different variants. First, as a feature extractor in addition to the text vectorization schemes’ features. We fed those features to various regression models to make a prediction value that represents the relevancy score between Arabic text units. Second, AraBERT is adopted as a pre-trained model, and its parameters are fine-tuned to estimate the relevancy scores between Arabic textual sentences. To evaluate the research results, we conducted several experiments to compare the use of the AraBERT model in its two variants. In terms of Mean Absolute Percentage Error (MAPE), the results show minor variance between AraBERT v0.2 as a feature extractor (21.7723) and the fine-tuned AraBERT v2 (21.8211). On the other hand, AraBERT v0.2-Large as a feature extractor outperforms the fine-tuned AraBERT v2 model on the used data set in terms of the coefficient of determination () values (0.014050,−0.032861), respectively.Keywords
Cite This Article
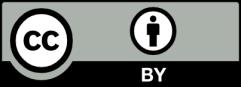
This work is licensed under a Creative Commons Attribution 4.0 International License , which permits unrestricted use, distribution, and reproduction in any medium, provided the original work is properly cited.