Open Access
ARTICLE
Self-Care Assessment for Daily Living Using Machine Learning Mechanism
1 Department of Computer Science, Air University, Islamabad, 44000, Pakistan
2 Department of Computer Science and Software Engineering, Al Ain University, Al Ain, 15551, UAE
3 Department of Computer Science, College of Computer, Qassim University, Buraydah, 51452, Saudi Arabia
4 Department of Humanities and Social Science, Al Ain University, Al Ain, 15551, UAE
5 Department of Computer Engineering, Korea Polytechnic University, 237 Sangidaehak-ro Siheung-si, Gyeonggi-do, 15073, Korea
* Corresponding Author: Jeongmin Park. Email:
Computers, Materials & Continua 2022, 72(1), 1747-1764. https://doi.org/10.32604/cmc.2022.025112
Received 12 November 2021; Accepted 11 January 2022; Issue published 24 February 2022
Abstract
Nowadays, activities of daily living (ADL) recognition system has been considered an important field of computer vision. Wearable and optical sensors are widely used to assess the daily living activities in healthy people and people with certain disorders. Although conventional ADL utilizes RGB optical sensors but an RGB-D camera with features of identifying depth (distance information) and visual cues has greatly enhanced the performance of activity recognition. In this paper, an RGB-D-based ADL recognition system has been presented. Initially, human silhouette has been extracted from the noisy background of RGB and depth images to track human movement in a scene. Based on these silhouettes, full body features and point based features have been extracted which are further optimized with probability based incremental learning (PBIL) algorithm. Finally, random forest classifier has been used to classify activities into different categories. The n-fold cross-validation scheme has been used to measure the viability of the proposed model on the RGBD-AC benchmark dataset and has achieved an accuracy of 92.71% over other state-of-the-art methodologies.Keywords
Cite This Article
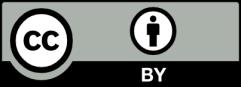
This work is licensed under a Creative Commons Attribution 4.0 International License , which permits unrestricted use, distribution, and reproduction in any medium, provided the original work is properly cited.