Open Access
ARTICLE
A Chaotic Oppositional Whale Optimisation Algorithm with Firefly Search for Medical Diagnostics
1 Singidunum University, 32 Danijelova Str., 11010, Belgrade, Serbia
2 Department of Applied Cybernetics, Faculty of Science, University of Hradec Králové, 50003, Hradec Králové, Czech Republic
* Corresponding Author: K. Venkatachalam. Email:
Computers, Materials & Continua 2022, 72(1), 959-982. https://doi.org/10.32604/cmc.2022.024989
Received 07 November 2021; Accepted 10 December 2021; Issue published 24 February 2022
Abstract
There is a growing interest in the study development of artificial intelligence and machine learning, especially regarding the support vector machine pattern classification method. This study proposes an enhanced implementation of the well-known whale optimisation algorithm, which combines chaotic and opposition-based learning strategies, which is adopted for hyper-parameter optimisation and feature selection machine learning challenges. The whale optimisation algorithm is a relatively recent addition to the group of swarm intelligence algorithms commonly used for optimisation. The Proposed improved whale optimisation algorithm was first tested for standard unconstrained CEC2017 benchmark suite and it was later adapted for simultaneous feature selection and support vector machine hyper-parameter tuning and validated for medical diagnostics by using breast cancer, diabetes, and erythemato-squamous dataset. The performance of the proposed model is compared with multiple competitive support vector machine models boosted with other metaheuristics, including another improved whale optimisation approach, particle swarm optimisation algorithm, bacterial foraging optimisation algorithms, and genetic algorithms. Results of the simulation show that the proposed model outperforms other competitors concerning the performance of classification and the selected subset feature size.Keywords
Cite This Article
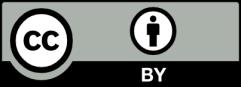