Open Access
ARTICLE
Historical Arabic Images Classification and Retrieval Using Siamese Deep Learning Model
1 Computer Science Department, Umm Al-Qura University, Makkah, Saudi Arabia
2 Computer Science Department, King Abdulaziz University, Jeddah, Saudi Arabia
3 Electrical Engineering Department, Faculty of Engineering at Shoubra, Benha University, Cairo, Egypt
4 Department of Information Systems and Technology, College of Computer Science and Engineering, University of Jeddah, Jeddah, Saudi Arabia
* Corresponding Author: Mashael M. Khayyat. Email:
Computers, Materials & Continua 2022, 72(1), 2109-2125. https://doi.org/10.32604/cmc.2022.024975
Received 06 November 2021; Accepted 11 January 2022; Issue published 24 February 2022
Abstract
Classifying the visual features in images to retrieve a specific image is a significant problem within the computer vision field especially when dealing with historical faded colored images. Thus, there were lots of efforts trying to automate the classification operation and retrieve similar images accurately. To reach this goal, we developed a VGG19 deep convolutional neural network to extract the visual features from the images automatically. Then, the distances among the extracted features vectors are measured and a similarity score is generated using a Siamese deep neural network. The Siamese model built and trained at first from scratch but, it didn't generated high evaluation metrices. Thus, we re-built it from VGG19 pre-trained deep learning model to generate higher evaluation metrices. Afterward, three different distance metrics combined with the Sigmoid activation function are experimented looking for the most accurate method for measuring the similarities among the retrieved images. Reaching that the highest evaluation parameters generated using the Cosine distance metric. Moreover, the Graphics Processing Unit (GPU) utilized to run the code instead of running it on the Central Processing Unit (CPU). This step optimized the execution further since it expedited both the training and the retrieval time efficiently. After extensive experimentation, we reached satisfactory solution recording 0.98 and 0.99 F-score for the classification and for the retrieval, respectively.Keywords
Cite This Article
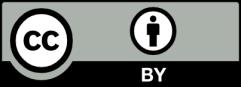
This work is licensed under a Creative Commons Attribution 4.0 International License , which permits unrestricted use, distribution, and reproduction in any medium, provided the original work is properly cited.