Open Access
ARTICLE
Optimized Load Balancing Technique for Software Defined Network
1 Department of Computer Science and Engineering, Chandigarh University, Mohali, Punjab, 140413, India
2 School of Computational Sciences, CHRIST (Deemed to be University), Ghaziabad, 201003, India
3 Department of Computer Science and Engineering, Sejong University, Seoul, 05006, Korea
4 Department of Automotive ICT Convergence Engineering, Daegu Catholic University, Gyeongsan, 38430, Korea
* Corresponding Author: Woong Cho. Email:
Computers, Materials & Continua 2022, 72(1), 1409-1426. https://doi.org/10.32604/cmc.2022.024970
Received 06 November 2021; Accepted 30 December 2021; Issue published 24 February 2022
Abstract
Software-defined networking is one of the progressive and prominent innovations in Information and Communications Technology. It mitigates the issues that our conventional network was experiencing. However, traffic data generated by various applications is increasing day by day. In addition, as an organization's digital transformation is accelerated, the amount of information to be processed inside the organization has increased explosively. It might be possible that a Software-Defined Network becomes a bottleneck and unavailable. Various models have been proposed in the literature to balance the load. However, most of the works consider only limited parameters and do not consider controller and transmission media loads. These loads also contribute to decreasing the performance of Software-Defined Networks. This work illustrates how a software-defined network can tackle the load at its software layer and give excellent results to distribute the load. We proposed a deep learning-dependent convolutional neural network-based load balancing technique to handle a software-defined network load. The simulation results show that the proposed model requires fewer resources as compared to existing machine learning-based load balancing techniques.Keywords
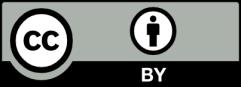