Open Access
ARTICLE
Enhancement of Biomass Material Characterization Images Using an Improved U-Net
1 College of Computer and Control Engineering, Qiqihar University, Qiqihar, 161006, China
2 College of Light Industry and Textile, Qiqihar University, Qiqihar, 161006, China
3 Elastic Block Store Performance Team, Amazon Web Services, Boston, 02210, USA
* Corresponding Author: Hong Zhao. Email:
Computers, Materials & Continua 2022, 72(1), 1515-1528. https://doi.org/10.32604/cmc.2022.024779
Received 31 October 2021; Accepted 05 January 2022; Issue published 24 February 2022
Abstract
For scanning electron microscopes with high resolution and a strong electric field, biomass materials under observation are prone to radiation damage from the electron beam. This results in blurred or non-viable images, which affect further observation of material microscopic morphology and characterization. Restoring blurred images to their original sharpness is still a challenging problem in image processing. Traditional methods can't effectively separate image context dependency and texture information, affect the effect of image enhancement and deblurring, and are prone to gradient disappearance during model training, resulting in great difficulty in model training. In this paper, we propose the use of an improved U-Net (U-shaped Convolutional Neural Network) to achieve image enhancement for biomass material characterization and restore blurred images to their original sharpness. The main work is as follows: use of depthwise separable convolution instead of standard convolution in U-Net to reduce model computation effort and parameters; embedding wavelet transform into the U-Net structure to separate image context and texture information, thereby improving image reconstruction quality; using dense multi-receptive field channel modules to extract image detail information, thereby better transmitting the image features and network gradients, and reduce the difficulty of training. The experiments show that the improved U-Net model proposed in this paper is suitable and effective for enhanced deblurring of biomass material characterization images. The PSNR (Peak Signal-to-noise Ratio) and SSIM (Structural Similarity) are enhanced as well.Keywords
Cite This Article
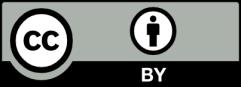
This work is licensed under a Creative Commons Attribution 4.0 International License , which permits unrestricted use, distribution, and reproduction in any medium, provided the original work is properly cited.