Open Access
ARTICLE
A Template Matching Based Feature Extraction for Activity Recognition
1 College of Computer and Information Sciences, Jouf University, Sakaka, Aljouf, 2014, Kingdom of Saudi Arabia
2 Department of Computer Science, COMSATS University Islamabad, Lahore Campus, Pakistan
* Corresponding Author: Muhammad Hameed Siddiqi. Email:
(This article belongs to the Special Issue: Face Image Analysis Using Deep Learning)
Computers, Materials & Continua 2022, 72(1), 611-634. https://doi.org/10.32604/cmc.2022.024760
Received 30 October 2021; Accepted 21 December 2021; Issue published 24 February 2022
Abstract
Human activity recognition (HAR) can play a vital role in the monitoring of human activities, particularly for healthcare conscious individuals. The accuracy of HAR systems is completely reliant on the extraction of prominent features. Existing methods find it very challenging to extract optimal features due to the dynamic nature of activities, thereby reducing recognition performance. In this paper, we propose a robust feature extraction method for HAR systems based on template matching. Essentially, in this method, we want to associate a template of an activity frame or sub-frame comprising the corresponding silhouette. In this regard, the template is placed on the frame pixels to calculate the equivalent number of pixels in the template correspondent those in the frame. This process is replicated for the whole frame, and the pixel is directed to the optimum match. The best count is estimated to be the pixel where the silhouette (provided via the template) presented inside the frame. In this way, the feature vector is generated. After feature vector generation, the hidden Markov model (HMM) has been utilized to label the incoming activity. We utilized different publicly available standard datasets for experiments. The proposed method achieved the best accuracy against existing state-of-the-art systems.Keywords
Cite This Article
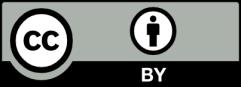
This work is licensed under a Creative Commons Attribution 4.0 International License , which permits unrestricted use, distribution, and reproduction in any medium, provided the original work is properly cited.