Open Access
ARTICLE
Artificial Intelligence-Based Fusion Model for Paddy Leaf Disease Detection and Classification
1 Department of Information Systems, College of Computer and Information Sciences, Prince Sultan University, Riyadh, 11586, Saudi Arabia
2 Department of Information Systems, College of Science and Arts, King Khalid University, Muhayel Aseer, 62529, Saudi Arabia
3 Department of Information Systems-Girls Section, King Khalid University, Muhayel Aseer, 62529, Saudi Arabia
4 Department of Natural and Applied Sciences, College of Community-Aflaj, Prince Sattam bin Abdulaziz University, Aflaj, 16828, Saudi Arabia
5 Computer Science Department, Community College-Girls Section, King Khalid University, Muhayel Aseer, 62529, Saudi Arabia
6 Department of Computer and Self Development, Preparatory Year Deanship, Prince Sattam bin Abdulaziz University, Al-Kharj, 16278, Saudi Arabia
* Corresponding Author: Manar Ahmed Hamza. Email:
Computers, Materials & Continua 2022, 72(1), 1391-1407. https://doi.org/10.32604/cmc.2022.024618
Received 25 October 2021; Accepted 05 January 2022; Issue published 24 February 2022
Abstract
In agriculture, rice plant disease diagnosis has become a challenging issue, and early identification of this disease can avoid huge loss incurred from less crop productivity. Some of the recently-developed computer vision and Deep Learning (DL) approaches can be commonly employed in designing effective models for rice plant disease detection and classification processes. With this motivation, the current research work devises an Efficient Deep Learning based Fusion Model for Rice Plant Disease (EDLFM-RPD) detection and classification. The aim of the proposed EDLFM-RPD technique is to detect and classify different kinds of rice plant diseases in a proficient manner. In addition, EDLFM-RPD technique involves median filtering-based preprocessing and K-means segmentation to determine the infected portions. The study also used a fusion of handcrafted Gray Level Co-occurrence Matrix (GLCM) and Inception-based deep features to derive the features. Finally, Salp Swarm Optimization with Fuzzy Support Vector Machine (FSVM) model is utilized for classification. In order to validate the enhanced outcomes of EDLFM-RPD technique, a series of simulations was conducted. The results were assessed under different measures. The obtained values infer the improved performance of EDLFM-RPD technique over recent approaches and achieved a maximum accuracy of 96.170%.Keywords
Cite This Article
A. S. Almasoud, A. Abdelmaboud, T. Abdalla Elfadil Eisa, M. Al Duhayyim, A. Abbas Hassan Elnour et al., "Artificial intelligence-based fusion model for paddy leaf disease detection and classification," Computers, Materials & Continua, vol. 72, no.1, pp. 1391–1407, 2022. https://doi.org/10.32604/cmc.2022.024618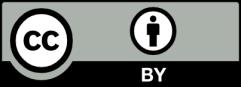