Open Access
ARTICLE
A Lightweight CNN Based on Transfer Learning for COVID-19 Diagnosis
1 Wuxi Research Institute, Nanjing University of Information Science & Technology, Wuxi, 214100, China
2 Engineering Research Center of Digital Forensics, Ministry of Education, Jiangsu Engineering Center of Network Monitoring, School of Computer and Software, Nanjing University of Information Science & Technology, Nanjing, 210044, China
3 Jiangsu Collaborative Innovation Center of Atmospheric Environment and Equipment Technology (CICAEET), Nanjing University of Information Science & Technology, Nanjing, 210044, China
4 School of Automation, Nanjing University of Information Science & Technology, Nanjing 210044 China
5 IT Fundamentals and Education Technologies Applications, University of Information Technology and Management in Rzeszow, Rzeszow Voivodeship, 100031, Poland
* Corresponding Author: Xiaorui Zhang. Email:
Computers, Materials & Continua 2022, 72(1), 1123-1137. https://doi.org/10.32604/cmc.2022.024589
Received 23 October 2021; Accepted 04 January 2022; Issue published 24 February 2022
Abstract
The key to preventing the COVID-19 is to diagnose patients quickly and accurately. Studies have shown that using Convolutional Neural Networks (CNN) to analyze chest Computed Tomography (CT) images is helpful for timely COVID-19 diagnosis. However, personal privacy issues, public chest CT data sets are relatively few, which has limited CNN's application to COVID-19 diagnosis. Also, many CNNs have complex structures and massive parameters. Even if equipped with the dedicated Graphics Processing Unit (GPU) for acceleration, it still takes a long time, which is not conductive to widespread application. To solve above problems, this paper proposes a lightweight CNN classification model based on transfer learning. Use the lightweight CNN MobileNetV2 as the backbone of the model to solve the shortage of hardware resources and computing power. In order to alleviate the problem of model overfitting caused by insufficient data set, transfer learning is used to train the model. The study first exploits the weight parameters trained on the ImageNet database to initialize the MobileNetV2 network, and then retrain the model based on the CT image data set provided by Kaggle. Experimental results on a computer equipped only with the Central Processing Unit (CPU) show that it consumes only 1.06 s on average to diagnose a chest CT image. Compared to other lightweight models, the proposed model has a higher classification accuracy and reliability while having a lightweight architecture and few parameters, which can be easily applied to computers without GPU acceleration. Code:github.com/ZhouJie-520/paper-codes.Keywords
Cite This Article
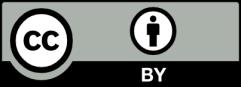
This work is licensed under a Creative Commons Attribution 4.0 International License , which permits unrestricted use, distribution, and reproduction in any medium, provided the original work is properly cited.