Open Access
ARTICLE
Malware Detection Using Decision Tree Based SVM Classifier for IoT
1 Department of Computer and Self Development, Preparatory Year Deanship, Prince Sattam bin Abdulaziz University, AlKharj, Saudi Arabia
2 Department of Computer Science, College of Science and Arts, King Khalid University, Mahayil Asir, Saudi Arabia
3 Department of Computer Science, College of Computer and Information Sciences, Prince Sultan University, Saudi Arabia
4 Department of Computer Science, College of Computing and Information System, Umm Al-Qura University, Saudi Arabia
5 Department of Natural and Applied Sciences, College of Community-Aflaj, Prince Sattam bin Abdulaziz University, Saudi Arabia
* Corresponding Author: Anwer Mustafa Hilal. Email:
Computers, Materials & Continua 2022, 72(1), 713-726. https://doi.org/10.32604/cmc.2022.024501
Received 20 October 2021; Accepted 25 November 2021; Issue published 24 February 2022
Abstract
The development in Information and Communication Technology has led to the evolution of new computing and communication environment. Technological revolution with Internet of Things (IoTs) has developed various applications in almost all domains from health care, education to entertainment with sensors and smart devices. One of the subsets of IoT is Internet of Medical things (IoMT) which connects medical devices, hardware and software applications through internet. IoMT enables secure wireless communication over the Internet to allow efficient analysis of medical data. With these smart advancements and exploitation of smart IoT devices in health care technology there increases threat and malware attacks during transmission of highly confidential medical data. This work proposes a scheme by integrating machine learning approach and block chain technology to detect malware during data transmission in IoMT. The proposed Machine Learning based Block Chain Technology malware detection scheme (MLBCT-Mdetect) is implemented in three steps namely: feature extraction, Classification and blockchain. Feature extraction is performed by calculating the weight of each feature and reduces the features with less weight. Support Vector Machine classifier is employed in the second step to classify the malware and benign nodes. Furthermore, third step uses blockchain to store details of the selected features which eventually improves the detection of malware with significant improvement in speed and accuracy. ML-BCT-Mdetect achieves higher accuracy with low false positive rate and higher True positive rate.Keywords
Cite This Article
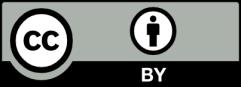
This work is licensed under a Creative Commons Attribution 4.0 International License , which permits unrestricted use, distribution, and reproduction in any medium, provided the original work is properly cited.