Open Access
ARTICLE
Machine Learning Enabled e-Learner Non-Verbal Behavior Detection in IoT Environment
1 Department of Information Systems, College of Science and Arts, King Khalid University, Mahayil Asir, Saudi Arabia
2 Department of Computer Science, College of Science & Art at Mahayil, King Khalid University, Saudi Arabia
3 Faculty of Computer and IT, Sana'a University, Sana'a, Yemen
4 Department of Natural and Applied Sciences, College of Community-Aflaj, Prince Sattam bin Abdulaziz University, Saudi Arabia
5 Department of Information Systems, College of Science and Arts-Girls Section, King Khalid University, Mahayil Asir, Saudi Arabia
6 Department of Computer and Self Development, Preparatory Year Deanship, Prince Sattam bin Abdulaziz University, AlKharj, Saudi Arabia
7 Department of Information Systems, College of Computer and Information Sciences, Princess Nourah bint Abdulrahman University, Saudi Arabia & Department of Mathematics, Faculty of Science, Cairo University, Giza, 12613, Egypt
* Corresponding Author: Manar Ahmed Hamza. Email:
Computers, Materials & Continua 2022, 72(1), 679-693. https://doi.org/10.32604/cmc.2022.024240
Received 10 October 2021; Accepted 22 November 2021; Issue published 24 February 2022
Abstract
Internet of Things (IoT) with e-learning is widely employed to collect data from various smart devices and share it with other ones for efficient e-learning applications. At the same time, machine learning (ML) and data mining approaches are presented for accomplishing prediction and classification processes. With this motivation, this study focuses on the design of intelligent machine learning enabled e-learner non-verbal behaviour detection (IML-ELNVBD) in IoT environment. The proposed IML-ELNVBD technique allows the IoT devices such as audio sensors, cameras, etc. which are then connected to the cloud server for further processing. In addition, the modelling and extraction of behaviour take place. Moreover, extreme learning machine sparse autoencoder (ELM-SAE) model is employed for the detection and classification of non-verbal behaviour. Finally, the Ant Colony Optimization (ACO) algorithm is utilized to properly tune the weight and bias parameters involved in the ELM-SAE model. In order to ensure the improved performance of the IML-ELNVBD model, a comprehensive simulation analysis is carried out and the results highlighted the betterment compared to the recent models.Keywords
Cite This Article
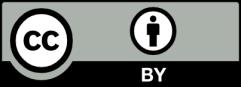
This work is licensed under a Creative Commons Attribution 4.0 International License , which permits unrestricted use, distribution, and reproduction in any medium, provided the original work is properly cited.