Open Access
ARTICLE
Hybrid Deep Learning Enabled Air Pollution Monitoring in ITS Environment
1 Department of Computer Science and Information Systems, College of Applied Sciences, AlMaarefa University, Ad Diriyah, Riyadh, 13713, Kingdom of Saudi Arabia
2 Department of Electronics and Communication Engineering, Kalasalingam Academy of Research and Education, Krishnankoil, 626128, India
3 Department of Computer Science, College of Computer Engineering and Sciences, Prince Sattam Bin Abdulaziz University, Al-Kharj 11942, Saudi Arabia
4 Department of Computer Science and Engineering, K. Ramakrishnan College of Technology, Tiruchirappalli, 621112, India
5 Department of Computer Applications, J. J College of Arts and Science (Autonomous), Affiliated to Bharathidasan University, Pudukkottai, 622422, India
6 Department of Entrepreneurship and Logistics, Plekhanov Russian University of Economics, 117997, Moscow, Russia
7 Department of Logistics, State University of Management, 109542, Moscow, Russia
* Corresponding Author: Kanagaraj Narayanasamy. Email:
Computers, Materials & Continua 2022, 72(1), 1157-1172. https://doi.org/10.32604/cmc.2022.024109
Received 05 October 2021; Accepted 09 December 2021; Issue published 24 February 2022
Abstract
Intelligent Transportation Systems (ITS) have become a vital part in improving human lives and modern economy. It aims at enhancing road safety and environmental quality. There is a tremendous increase observed in the number of vehicles in recent years, owing to increasing population. Each vehicle has its own individual emission rate; however, the issue arises when the emission rate crosses a standard value. Owing to the technological advances made in Artificial Intelligence (AI) techniques, it is easy to leverage it to develop prediction approaches so as to monitor and control air pollution. The current research paper presents Oppositional Shark Shell Optimization with Hybrid Deep Learning Model for Air Pollution Monitoring (OSSO-HDLAPM) in ITS environment. The proposed OSSO-HDLAPM technique includes a set of sensors embedded in vehicles to measure the level of pollutants. In addition, hybridized Convolution Neural Network with Long Short-Term Memory (HCNN-LSTM) model is used to predict pollutant level based on the data attained earlier by the sensors. In HCNN-LSTM model, the hyperparameters are selected and optimized using OSSO algorithm. In order to validate the performance of the proposed OSSO-HDLAPM technique, a series of experiments was conducted and the obtained results showcase the superior performance of OSSO-HDLAPM technique under different evaluation parameters.Keywords
Cite This Article
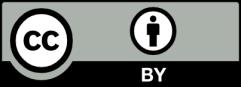
This work is licensed under a Creative Commons Attribution 4.0 International License , which permits unrestricted use, distribution, and reproduction in any medium, provided the original work is properly cited.