Open Access
ARTICLE
A Two-Tier Framework Based on GoogLeNet and YOLOv3 Models for Tumor Detection in MRI
1 Department of Software, Sejong University, Seoul, 05006, Korea
2 Institute of Computer Sciences & Information Technology, The University of Agriculture, Peshawar, 25130, Pakistan
3 College of Technological Innovation, Zayed University, Dubai, 19282, UAE
4 Department of Software, Korea Aerospace University, Seoul, 10540, Korea
5 Faculty of Computer Science and Engineering, Galala University, Suez, 435611, Egypt
6 Information Systems Department, Faculty of Computers and Artificial Intelligence, Benha University, Banha, 13518, Egypt
7 Chitkara University Institute of Engineering & Technology, Chitkara University, Rajpura, 140401, India
* Corresponding Author: Dongho Song. Email:
(This article belongs to the Special Issue: Machine Learning Empowered Secure Computing for Intelligent Systems)
Computers, Materials & Continua 2022, 72(1), 73-92. https://doi.org/10.32604/cmc.2022.024103
Received 04 October 2021; Accepted 18 November 2021; Issue published 24 February 2022
Abstract
Medical Image Analysis (MIA) is one of the active research areas in computer vision, where brain tumor detection is the most investigated domain among researchers due to its deadly nature. Brain tumor detection in magnetic resonance imaging (MRI) assists radiologists for better analysis about the exact size and location of the tumor. However, the existing systems may not efficiently classify the human brain tumors with significantly higher accuracies. In addition, smart and easily implementable approaches are unavailable in 2D and 3D medical images, which is the main problem in detecting the tumor. In this paper, we investigate various deep learning models for the detection and localization of the tumor in MRI. A novel two-tier framework is proposed where the first tire classifies normal and tumor MRI followed by tumor regions localization in the second tire. Furthermore, in this paper, we introduce a well-annotated dataset comprised of tumor and normal images. The experimental results demonstrate the effectiveness of the proposed framework by achieving 97% accuracy using GoogLeNet on the proposed dataset for classification and 83% for localization tasks after fine-tuning the pre-trained you only look once (YOLO) v3 model.Keywords
Cite This Article
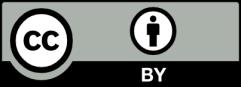
This work is licensed under a Creative Commons Attribution 4.0 International License , which permits unrestricted use, distribution, and reproduction in any medium, provided the original work is properly cited.