Open Access
ARTICLE
Prognostic Kalman Filter Based Bayesian Learning Model for Data Accuracy Prediction
1 Department of ECE, College of Engineering and Technology, SRM Institute of Science and Technology, Vadapalani, Chennai, 600026, India
2 Department of CSE, Birla Institute of Applied Sciences (BIAS), Bhimtal, Uttarakhand, 263136, India
3 Division of Applied Mathematics, Wonkwang University, 460, Iksan-Daero, Iksan-Si, Jeonbuk, 54538, Korea
4 School of Computer Science and Engineering, Vellore Institute of Technology (VIT), Chennai, 600127, India
5 Department of Mathematics, Tamralipta Mahavidyalaya, West Bengal, 721636, India
6 School of Computer Science and Engineering, Center for Cyber Physical Systems, Vellore Institute of Technology (VIT), Chennai, 600127, India
7 Indian Institute of Information Technology (IIIT), Lucknow, Uttar Pradesh, 226002, India
8 Department of Computer Science and Engineering, Saveetha School of Engineering, SIMATS, Chennai, 602105, India
* Corresponding Author: Jeong Gon Lee. Email:
(This article belongs to the Special Issue: Innovative Technology For Machine Intelligence)
Computers, Materials & Continua 2022, 72(1), 243-259. https://doi.org/10.32604/cmc.2022.023864
Received 24 September 2021; Accepted 07 December 2021; Issue published 24 February 2022
Abstract
Data is always a crucial issue of concern especially during its prediction and computation in digital revolution. This paper exactly helps in providing efficient learning mechanism for accurate predictability and reducing redundant data communication. It also discusses the Bayesian analysis that finds the conditional probability of at least two parametric based predictions for the data. The paper presents a method for improving the performance of Bayesian classification using the combination of Kalman Filter and K-means. The method is applied on a small dataset just for establishing the fact that the proposed algorithm can reduce the time for computing the clusters from data. The proposed Bayesian learning probabilistic model is used to check the statistical noise and other inaccuracies using unknown variables. This scenario is being implemented using efficient machine learning algorithm to perpetuate the Bayesian probabilistic approach. It also demonstrates the generative function for Kalman-filer based prediction model and its observations. This paper implements the algorithm using open source platform of Python and efficiently integrates all different modules to piece of code via Common Platform Enumeration (CPE) for Python.Keywords
Cite This Article
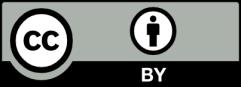
This work is licensed under a Creative Commons Attribution 4.0 International License , which permits unrestricted use, distribution, and reproduction in any medium, provided the original work is properly cited.