Open Access
ARTICLE
Identification and Classification of Crowd Activities
1 Department of Information Technology, Faculty of Computers and Information, Mansoura University, Egypt
2 Department of Computer Science, College of Computer Engineering and Sciences, Prince Sattam Bin Abdulaziz University, Al-Kharj, 11942, Saudi Arabia
3 Department of Information Systems, Faculty of Computers and Artificial Intelligence, Benha University, Egypt
4 Faculty of Computer Science and Engineering, Galala University, 435611, Suez, Egypt
5 Department of Computer Science, Faculty of Media Engineering and Technology, German University, Egypt
* Corresponding Author: Ahmed O. Aseeri. Email:
Computers, Materials & Continua 2022, 72(1), 815-832. https://doi.org/10.32604/cmc.2022.023852
Received 24 September 2021; Accepted 24 December 2021; Issue published 24 February 2022
Abstract
The identification and classification of collective people's activities are gaining momentum as significant themes in machine learning, with many potential applications emerging. The need for representation of collective human behavior is especially crucial in applications such as assessing security conditions and preventing crowd congestion. This paper investigates the capability of deep neural network (DNN) algorithms to achieve our carefully engineered pipeline for crowd analysis. It includes three principal stages that cover crowd analysis challenges. First, individual's detection is represented using the You Only Look Once (YOLO) model for human detection and Kalman filter for multiple human tracking; Second, the density map and crowd counting of a certain location are generated using bounding boxes from a human detector; and Finally, in order to classify normal or abnormal crowds, individual activities are identified with pose estimation. The proposed system successfully achieves designing an effective collective representation of the crowd given the individuals in addition to introducing a significant change of crowd in terms of activities change. Experimental results on MOT20 and SDHA datasets demonstrate that the proposed system is robust and efficient. The framework achieves an improved performance of recognition and detection people with a mean average precision of 99.0%, a real-time speed of 0.6 ms non-maximum suppression (NMS) per image for the SDHA dataset, and 95.3% mean average precision for MOT20 with 1.5 ms NMS per image.Keywords
Cite This Article
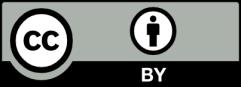