Open Access
ARTICLE
COVID-19 Severity Prediction Using Enhanced Whale with Salp Swarm Feature Classification
1 Singidunum University, Belgrade, 11000, Serbia
2 Department of Electronics and Communication Engineering, Amrita School of Engineering, Amrita Vishwa Vidyapeetham, Coimbatore, 641112, India
3 Department of Applied Cybernetics, Faculty of Science, University of Hradec Králové, Hradec Králové, 50003, Czech Republic
* Corresponding Author: Nebojsa Bacanin. Email:
Computers, Materials & Continua 2022, 72(1), 1685-1698. https://doi.org/10.32604/cmc.2022.023418
Received 07 September 2021; Accepted 06 January 2022; Issue published 24 February 2022
Abstract
Computerized tomography (CT) scans and X-rays play an important role in the diagnosis of COVID-19 and pneumonia. On the basis of the image analysis results of chest CT and X-rays, the severity of lung infection is monitored using a tool. Many researchers have done in diagnosis of lung infection in an accurate and efficient takes lot of time and inefficient. To overcome these issues, our proposed study implements four cascaded stages. First, for pre-processing, a mean filter is used. Second, texture feature extraction uses principal component analysis (PCA). Third, a modified whale optimization algorithm is used (MWOA) for a feature selection algorithm. The severity of lung infection is detected on the basis of age group. Fourth, image classification is done by using the proposed MWOA with the salp swarm algorithm (MWOA-SSA). MWOA-SSA has an accuracy of 97%, whereas PCA and MWOA have accuracies of 81% and 86%. The sensitivity rate of the MWOA-SSA algorithm is better that of than PCA (84.4%) and MWOA (95.2%). MWOA-SSA outperforms other algorithms with a specificity of 97.8%. This proposed method improves the effective classification of lung affected images from large datasets.Keywords
Cite This Article
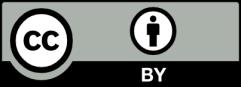