Open Access
ARTICLE
Effective Frameworks Based on Infinite Mixture Model for Real-World Applications
1 Department of Computer Sciences, College of Computer and Information Sciences, Princess Nourah bint Abdulrahman University, P.O.Box 84428, Riyadh 11671, Saudi Arabia
2 College of Computers and Information Technology, Taif University, Taif, 21944, Saudi Arabia
3 The Concordia Institute for Information Systems Engineering (CIISE), Concordia University, Montreal, QC H3G 1T7, Canada
* Corresponding Author: Sami Bourouis. Email:
(This article belongs to the Special Issue: Recent Advances in Metaheuristic Techniques and Their Real-World Applications)
Computers, Materials & Continua 2022, 72(1), 1139-1156. https://doi.org/10.32604/cmc.2022.022959
Received 24 August 2021; Accepted 01 December 2021; Issue published 24 February 2022
Abstract
Interest in automated data classification and identification systems has increased over the past years in conjunction with the high demand for artificial intelligence and security applications. In particular, recognizing human activities with accurate results have become a topic of high interest. Although the current tools have reached remarkable successes, it is still a challenging problem due to various uncontrolled environments and conditions. In this paper two statistical frameworks based on nonparametric hierarchical Bayesian models and Gamma distribution are proposed to solve some real-world applications. In particular, two nonparametric hierarchical Bayesian models based on Dirichlet process and Pitman-Yor process are developed. These models are then applied to address the problem of modelling grouped data where observations are organized into groups and these groups are statistically linked by sharing mixture components. The choice of the Gamma mixtures is motivated by its flexibility for modelling heavy-tailed distributions. In addition, deploying the Dirichlet process prior is justified by its advantage of automatically finding the right number of components and providing nice properties. Moreover, a learning step via variational Bayesian setting is presented in a flexible way. The priors over the parameters are selected appropriately and the posteriors are approximated effectively in a closed form. Experimental results based on a real-life applications that concerns texture classification and human actions recognition show the capabilities and effectiveness of the proposed framework.Keywords
Cite This Article
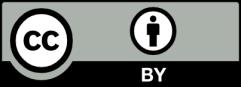
This work is licensed under a Creative Commons Attribution 4.0 International License , which permits unrestricted use, distribution, and reproduction in any medium, provided the original work is properly cited.